Doctoral defense in Electrical and Computer Engineering - Burkni Pálsson
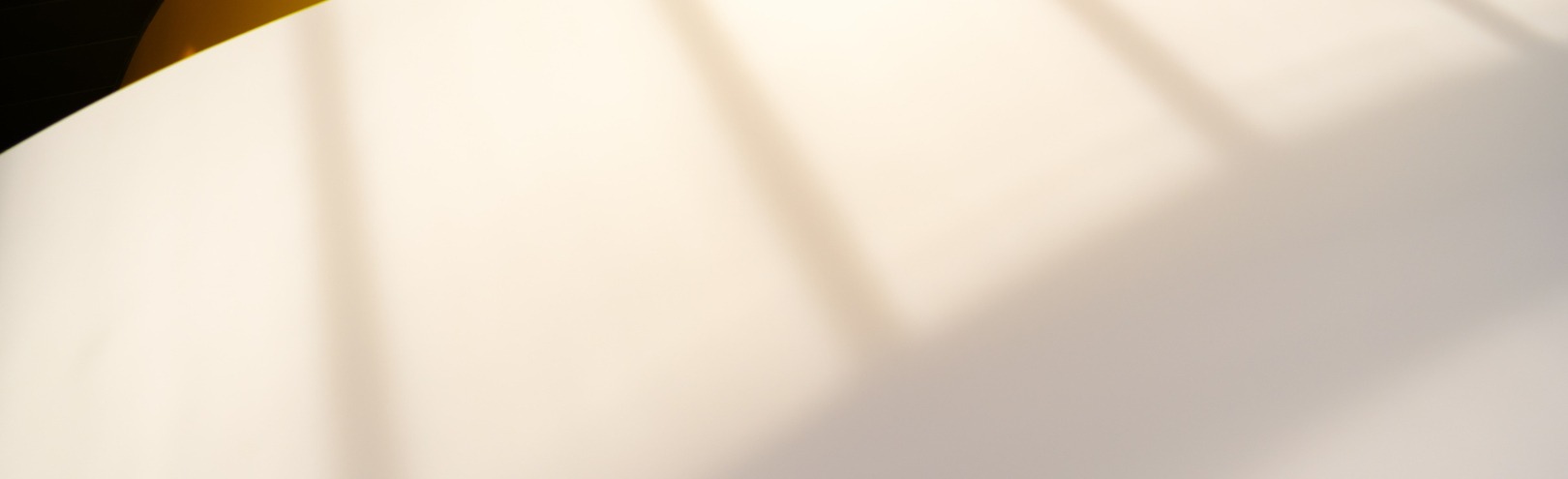
Aðalbygging
The Aula
Live stream link:
https://livestream.com/hi/doktorsvornburknipalsson
Doctoral candidate: Burkni Pálsson
Dissertation title: Blind Hyperspectral Unmixing Using Autoencoders
Opponents: Dr. Jie Chen, Professor at the Northwestern Polytechnical University, China and Dr. Ioannis Schizas, Associate Professor at the University of Texas at Arlington, Texas, USA.
Advisors: Dr. Jóhannes R. Sveinsson and Dr. Magnús Örn Úlfarsson, Professors at the Faculty of Electrical and Computer Engineering, University of Iceland.
Other members of Doctoral Committee: Dr. Jocelyn Chanussot, Professor at the Department for Signals and Images, Grenoble Institute of Technology, France.
Chair of Ceremony: Dr. Lotta María Ellingsen, Associate Professor and Head of Faculty at the Faculty of Electrical and Computer Engineering, University of Iceland.
Abstract
In remote sensing, hyperspectral images are images of the surface of the earth which are aquired by airborne or spaceborne sensors and have tens or hundres of channels, where each channel covers a narrow band of frequencies of light. Every pixel is thus a spectrum and this makes it possible to determine which pure materials are present in the image. Because hyperspectral images have low spatial resolution, most pixels contain more than one pure material. Hyperspectral umixing is the procedure of determining the spectra of pure materials in the image and the abundance of each material in every pixel. The subject of the thesis is blind hyperspectral unmixing using deep learning based autoencoders. Two methods based on autoencoders are proposed and analyzed. Both methods seek to exploit the spatial correlations in the hyperspectral images to improve the performance. One by using multitask learning to simultaneously unmix a neighbourhood of pixels while the other by using a convolutional autoencoder. This increases the consistency and robustness of the methods. In addition, a review of the various autoencoder methods in the literature is given along with a detailed discussion of different types of autoencoders. The thesis concludes by a critical comparison of eleven different autoencoder based methods. Ablation experiments are performed to answer the question of why autoencoders are so effective in blind hyperspectral unmixing, and an opinion is given on what the future in autoencoder unmixing holds.
About the doctoral candidate
Burkni Pálsson was born in Reykjavík in 1974. He graduated with degrees in both mathematics and physics in 1999 and with B.Sc degree in energy and environmental technology from the same school in 2012. In 2017, Burkni started his master and PhD studies in electrical and computer engineering at the University of Iceland and he graduated with a M.Sc. degree in 2020. Burkni has been the CTO of Geosilica Iceland since 2012, and he taught numerous courses in engineering technology at the University of Iceland from 2013 to 2018. Burkni’s research interests are signal processing, remote sensing, machine learning and deep learning.
Burkni Pálsson defends his Ph.D. thesis at the Faculty of Electrical and Computer Engineering in the University of Iceland. The title is Blind Hyperspectral Unmixing Using Autoencoders.
