Doctoral defense in Computational Engineering - Rocco Sedona
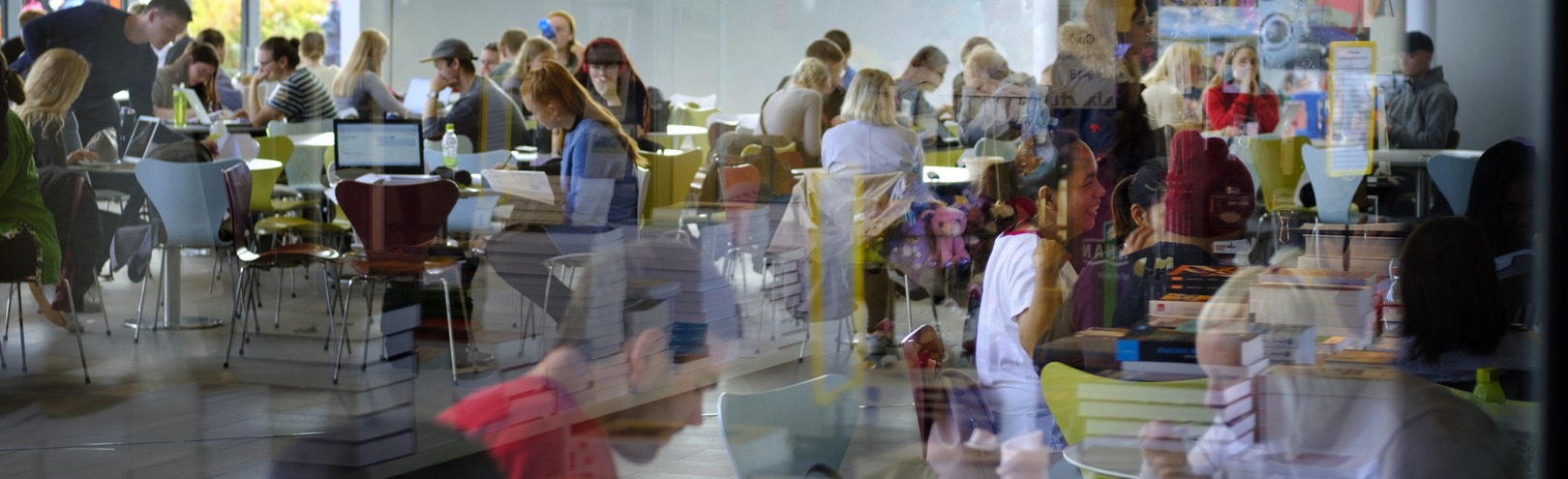
Aðalbygging
The Aula
Doctoral candidate: Rocco Sedona
Dissertation title: Scalable Deep Learning for Remote Sensing with High Performance Computing
Advisor: Dr. Morris Riedel, Professor at Faculty of Industrial Engineering, Mechanical Engineering and Computer Science, University of Iceland.
Also in the doctoral committee: Prof. Gabriele Cavallaro, University of Iceland and Forschungszentrum Juelich - Juelich Supercomputing Centre & Prof. Matthias Book, University of Iceland.
Opponents: Dr. Steven Reising, Professor of Electrical and Computer Engineering, Colorado State University, USA & Dr. Sergio Bernabé, Assistant Professor of Computer Science, Universidad Complutense, Madrid, Spain.
Chair of Ceremony: Dr. Rúnar Unnþórsson, Professor and Head of Faculty of Industrial Engineering, Mechanical Engineering and Computer Science.
Abstract
The advancement of Remote Sensing (RS) missions in the last decades has greatly increased the volume of data that is continuously acquired and made available to the end users, who can utilize them in a variety of Earth Observation (EO) appli- cations. Land Cover (LC) maps play a key role in the monitoring of the Earth’s surface, providing scientists and policymakers with an accurate view on the evolu- tion of the landscape, helping them to provide answers to pressing questions, from an efficient planning of the resources to resilience to climate change. Thanks to the usage of classical Machine Learning (ML) and more recently of Deep Learning (DL) methods, the information content of the RS can be exploited to an unprecedented extent, fostering research, development and deployment of frameworks to address open challenges for EO applications, including LC classification. However, the in- creased size of the datasets needed to train State of the Art (SotA) DL models and the need to utilize them at scale results in an increased time to deployment, which can hinder their effective utilization. The adoption of strategies for Distibuted Deep Learning (DDL) on High Performance Computing (HPC) systems offers the opportunity to speed-up the training of the models, allowing the researcher a faster development and reducing the time to value. As space agencies operate a variety of missions, data acquired by different sensors can be used to virtually increase the temporal resolution at which a certain area is observed, with potential benefits to the accuracy of the ML/DL models. The thesis objectives are formulated consider- ing these premises, and were investigated with a combination of methodologies to exploit the dedicated resources on HPC systems, contributing to answering open questions on the adoption of DDL methods for EO applications and to familiarize the RS community with such approaches, which can be of great value.
About the doctoral candidate
Rocco is a PhD candidate from Venice, Italy who obtained his Master's Degree in Information Engineering from the University of Trento in 2019. Currently, he is a PhD candidate at the University of Iceland and at the Jülich Supercomputing Centre in Germany. His research interests primarily lie in the field of Deep Learning and its application to Remote Sensing data. Additionally, he specializes in Distributed Deep Learning on High-Performance Computing systems, an area of study that he has been actively engaged in since 2019.
Rocco Sedona defends his Ph.D. thesis in computational engineering from the Faculty of Industrial Engineering, Mechanical Engineering and Computer Science at the University of Iceland.
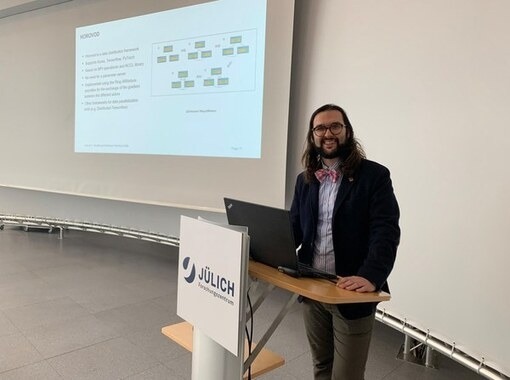