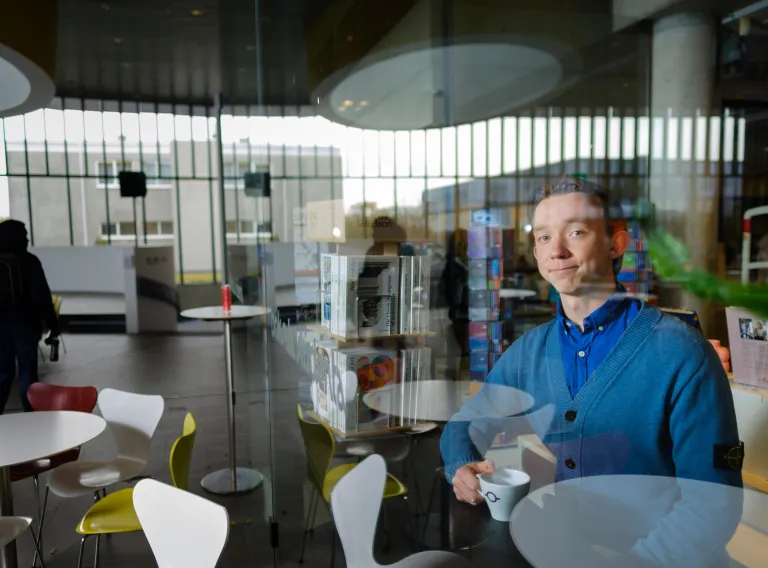
Changes may occur for the autumn semester in August and September and for the spring semester in December and January. You will find your final timetable in Ugla when the studies start.
Note! This timetable is not suitable for planning your work schedule if you are a part-time employee.