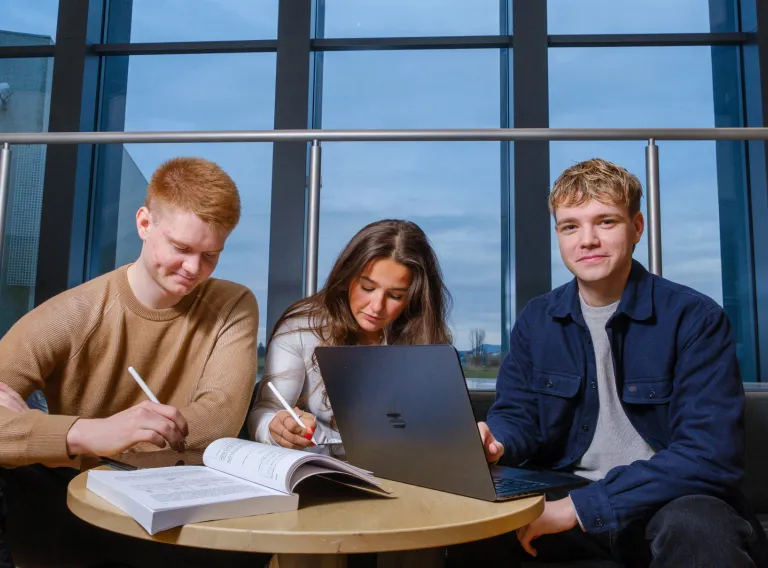
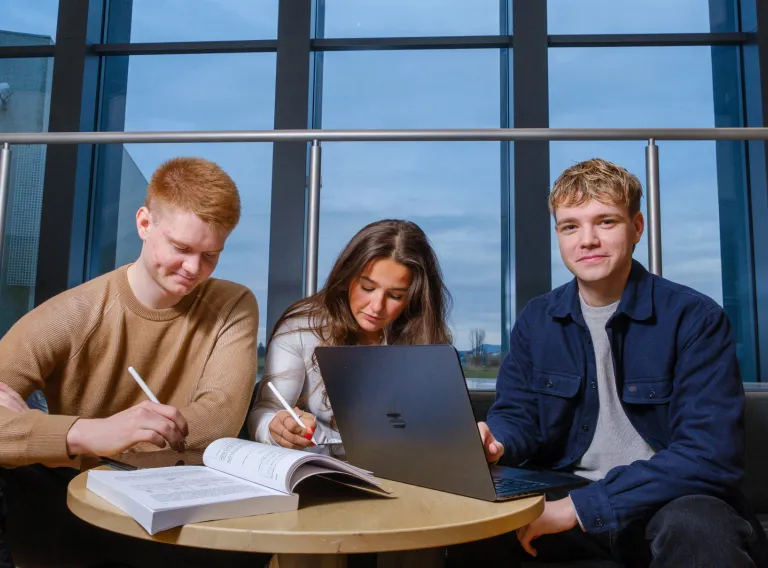
- Are you interested in statistics, data analysis and risk analysis?
- Are you interested in risk factors affecting health, disease distribution, preventative measures and interventions?
- Would you like to learn the methodology for health research, how to implement studies and interpret them?
- Would you like to learn how to carry out statistical processing on studies in the many different fields of biomedical science?
- Would you like to develop a strong foundation in statistics and statistic software such as R as used in research and data processing?
The MS in epidemiology and biostatistics is an interdisciplinary programme offered jointly by all schools at UI. The programme focuses on epidemiological methods and statistics/biostatistic and the application of these methods in population-based studies in the fields of public health and health sciences.
Elective courses from multiple UI faculties and the scope of the thesis project allow students to tailor the programme to suit their interests.
Main objectives
The programme aims to provide students with practical knowledge and skills in population-based studies and train them to apply these studies and interpret research results in the field of public health and health sciences. An understanding of this kind of research is an essential foundation for policymaking in health promotion and preventative measures.
Programme structure
The programme is 120 ECTS and is organised as two years of full-time study or three years of part-time study.
The programme is made up of 40 ECTS of mandatory courses, a final project worth either 30 or 60 ECTS, and 20-50 ECTS of restricted and free elective courses.
Specialisations
Students choose between the following specialisations in the first semester of the programme:
- Biostatistics: This specialisation provides students with in-depth training in the application of statistics in population-based studies in biology, genetics, public health and health sciences. It also focuses on a strong general foundation in statistics and data processing.
- Epidemiology: This specialisation provides practical knowledge in epidemiological methods and training in how to apply them in health science research. It also focuses on a solid foundation in statistics and the interpretation of research results.
Organisation of teaching
Mandatory courses and many restricted electives are completed through face-to-face learning and in some cases attendance is compulsory. The programme is taught in Icelandic or English.
Other
Completing the programme allows a student to apply for doctoral studies.
Applicants must hold an undergraduate degree (BS, BA, or comparable) from an accredited college/university. A specific field of study is not required, but our students are expected to have a solid background in methodology. Students who choose to specialize in biostatistics are also expected to have completed courses in mathematical analysis, linear algebra, as well as in probability and statistics. International applicants for whom English is not their first language must submit a TOEFL or IELTS score as proof of English proficiency. Minimum scores accepted are 79 on the TOEFL internet-based test or a IELTS score of 6,5.
Applicants are evaluated for entrance based on the following criteria:
1. Grade point average (in general a GPA equivalent to the Icelandic 7,25 (first) is a prerequisite for admissions). - Performance in specific courses, such as methodology and statistics.
2. Statement of purpose and objectives.
Students must complete 120 credits, divided as follows: 40 credits of mandatory courses, a 30 or 60 credit research thesis, as well as required and free elective courses.
- CV
- Statement of purpose
- Reference 1, Name and email
- Reference 2, Name and email
- Certified copies of diplomas and transcripts
- Use this form for the Personal statement
- Proof of English proficiency
Further information on supporting documents can be found here
Interdisciplinary programme.
- The Centre of Public Health Sciences coordinates the programme.
Programme structure
Check below to see how the programme is structured.
- First year
- Fall
- Applied Linear Statistical Models
- Biostatistics II (Clinical Prediction Models )
- Orientation seminar: public health, epidemiology and biostatistics
- Epidemiology - a quantitative methodology
- R Programming
- Spring 1
- Biostatistics III (Survival analysis)
- Not taught this semesterStatistical Consulting
- The Scientific Process: Ethics, Communication and Practicalities
Applied Linear Statistical Models (STÆ312M)
The course focuses on simple and multiple linear regression as well as analysis of variance (ANOVA), analysis of covariance (ANCOVA) and binomial regression. The course is a natural continuation of a typical introductory course in statistics taught in various departments of the university.
We will discuss methods for estimating parameters in linear models, how to construct confidence intervals and test hypotheses for the parameters, which assumptions need to hold for applying the models and what to do when they are not met.
Students will work on projects using the statistical software R.
Biostatistics II (Clinical Prediction Models ) (LÝÐ301F)
This course is a continuation of Biostatistics I and constitutes a practical guide to statistical analyses of student's own research projects. The course covers the following topics. Estimation of relative risk/odds ratios and adjusted estimation of relative risk/odds ratios, correlation and simple linear regression, multiple linear regression and logistic regression. The course is based on lectures and practical sessions using R for statistical analyses.
Orientation seminar: public health, epidemiology and biostatistics (LÝÐ108F)
This is a preparatory course for students in these interdisciplinary and research based studies. The course covers various practical issues, methods, study planning, refernce search, and scientific literacy e.g.. Students also aquire basic training in the statistical program R.
Epidemiology - a quantitative methodology (LÝÐ107F)
The course is an introduction to epidemiological research methods and causal inference. An overview is provided on measure of disease occurrence, measures of outcome (relative risks), and study design (experiments, intervention studies, cohort studies and case-control studies). Emphasis is on systematic errors and on methods to avoid such errors in planning (study design) and in data analyses. Students get training in reviewing epidemiological studies.
R Programming (MAS102M)
Students will perform traditional statistical analysis on real data sets. Special focus will be on regression methods, including multiple regression analysis. Students will apply sophisticated methods of graphical representation and automatic reporting. Students will hand in a projects where they apply the above mentioned methods on real datasets in order to answer research questions
Biostatistics III (Survival analysis) (LÝÐ079F)
The course covers methods for analysis of cohort studies using methods for time to event or survival analysis. It is based on the course Biostat III – Survival analysis for epidemiologists in R at the Karolinska Institutet: See (https://biostat3.net/index.html): "Topics covered include methods for estimating patient survival (life table and Kaplan-Meier methods), comparing survival between patient subgroups (log-rank test), and modelling survival (primarily Poisson regression, Cox proportional hazards model and flexible parametric models). The course addresses the concept of 'time' as a potential confounder or effect modifier and approaches to defining 'time' (e.g., time since entry, attained age, calendar time). The course will emphasise the basic concepts of statistical modelling in epidemiology, such as controlling for confounding and assessing effect modification."
Statistical Consulting (MAS201M)
Participants in the course will obtain training in practical statistics as used when providing general statistical counselling. The participants will be introduced to actual statistical projects by assisting students in various departments within the university. The participants will report on the projects in class, discuss options for solving the projects and subsequently assist the students with analyses using R and interpretation of results.
The Scientific Process: Ethics, Communication and Practicalities (LÝÐ202F)
The course constitutes a practical guide to the preparation of a health-related research study. Modules include: reference search and handling, development of hypotheses, creation of a systematic critical review within chosen field of research, development and presentation of research proposals.
The course is for graduate students who have chosen a field/research question for their dissertation project.
Students in MPH Public Health Sciences and MS Epidemiology and Biostatistics must pass the course before starting work on their final thesis.
- Second year
- Whole year courses
- Research Training in Public Health Sciences
- Fall
- Thesis in Epidemiology and Biostatistics
- Spring 1
- Thesis in Epidemiology and Biostatistics
Research Training in Public Health Sciences (LÝÐ098F)
The aim of the course is for students to gain training in methods and insight into the implementation of a specific research project in public health. Students get to know the theoretical background of the research they are working on and are trained to participate in a defined part of it, but the topics depend on the needs of the research project that is assigned. An example would be e.g. participation in the preparation of a research project and various work related to data collection and data processing (cleaning and/or analysis). Students will also get to know the different aspects of the research project under the guidance of the scientific staff leading the research and in collaboration with the research team.
Students attend regular meetings with the researchers and supervisors of the course. The research training is planned specifically for each student with regard to the content and progress of the research, the supervisor and the student's background. Study space is limited by the research projects that are ongoing at the Center for Public Health Sciences at any given time, and students apply for registration for the course at the program office.
Thesis in Epidemiology and Biostatistics (LÝÐ060L)
The MS program in Epidemiology and Biostatistics is a 120 credit post-graduate and cross-disciplinary program, including a 30 or 60 credit research thesis. Projects that have been/could be submitted for publication can be awarded 60 credits. During the first semester students develop their research questions and choose a thesis advisor. A complete research proposal is presented at the end of the second semester. Departmental registration is determined by the students' area of interest and host faculty of their main advisor.
The objective of the MS thesis is to provide training in constructing, organising, developing and conducting a research or developmental project in the public health sciences and an understanding of the technical limits, regulations, ethics and laws that must be observed in such work. Students should be able to define and present research questions and propose original hypotheses.
Thesis in Epidemiology and Biostatistics (LÝÐ060L)
The MS program in Epidemiology and Biostatistics is a 120 credit post-graduate and cross-disciplinary program, including a 30 or 60 credit research thesis. Projects that have been/could be submitted for publication can be awarded 60 credits. During the first semester students develop their research questions and choose a thesis advisor. A complete research proposal is presented at the end of the second semester. Departmental registration is determined by the students' area of interest and host faculty of their main advisor.
The objective of the MS thesis is to provide training in constructing, organising, developing and conducting a research or developmental project in the public health sciences and an understanding of the technical limits, regulations, ethics and laws that must be observed in such work. Students should be able to define and present research questions and propose original hypotheses.
- Year unspecified
- Fall
- Public Health: Science, Politics, Prevention
- Bayesian Data Analysis
- Mixed Linear Models
- Topics in Epidemiology (Epidemiology III)
- Theoretical Statistics
- Latent variable models I
- Not taught this semesterBiometry
- Construction of self report scales
- Not taught this semesterHuman Genetics
- Time Series Analysis
- Not taught this semesterTheory of linear models
- Computing and Calculus for Applied Statistics
- Spring 1
- Epidemiologic Methods (Epidemiology II)
- Random Effects Models
- Not taught this semesterStochastic Processes
- Trauma and its impact on health
- Applied data analysis
- Applied multivariable regression and data analysis
- Social science statistics: Regression analysis
- Genomics and bioinformatics
- Molecular Genetics
- Introduction to Measure-Theoretic Probability
- Genomics and bioinformatics
- Algorithms in Bioinformatics
Public Health: Science, Politics, Prevention (LÝÐ101F)
The course provides an overview of definitions, history, aims, legislation, methods and ethical considerations in public health and public health sciences. The course lays emphasis on global public health as well as on the Icelandic health care system, its administration and funding in comparison with health care systems in other nations. An overview is provided on Icelandic and international databases on health and disease and possibilities for their utilization in research and policy making for health promotion. In addition, current public health issues at each time are emphasized.
Bayesian Data Analysis (STÆ529M)
Goal: To train students in applying methods of Bayesian statistics for analysis of data. Topics: Theory of Bayesian inference, prior distributions, data distributions and posterior distributions. Bayesian inference for parameters of univariate and multivariate distributions: binomial; normal; Poisson; exponential; multivariate normal; multinomial. Model checking and model comparison: Bayesian p-values; deviance information criterion (DIC). Bayesian computation: Markov chain Monte Carlo (MCMC) methods; the Gibbs sampler; the Metropolis-Hastings algorithm; convergence diagnostistics. Linear models: normal linear models; hierarchical linear models; generalized linear models. Emphasis on data analysis using software, e.g. Matlab and R.
Mixed Linear Models (LÝÐ0A1F)
The course is about the theory and application of random effects, or linear mixed models, and related models for correlated response variables. The course will cover methods for continuous and approximately normally distributed variables. A statistical model for such data has to describe both the expected value and the covariance between observations. The theory extends the theory of general linear models. Special software is needed for such an analysis and the necessary packages are provided in R, STATA, and SAS. The application will be based on R but other programs will be introduced for comparison.
The course will be taught between beginning of September and end of November, meeting once a week. The teaching will be in a flipped class manner. All the material (both notes and lectures) are online (see below) and it is expected that students will have viewed the material before class. In class the theory will be discussed and then students are expected to work on the application on their own computer.
Topics in Epidemiology (Epidemiology III) (LÝÐ097F)
The aim of the course is to increase students' understanding of different areas within epidemiology, provide an introduction to area-specific methods, and to enhance students' ability to interpret results and assess the quality of scientific research in epidemiology.
The course will cover 4-6 specific areas or topics within epidemiology. Examples include perinatal, nutritional, pharmacological, and infectious disease epidemiology; featured topics may vary from year to year.
Theoretical Statistics (STÆ313M)
Likelihood, Sufficient Statistic, Sufficiency Principle, Nuisance Parameter, Conditioning Principle, Invariance Principle, Likelihood Theory. Hypothesis Testing, Simple and Composite Hypothesis, The Neyman-Pearson Lemma, Power, UMP-Test, Invariant Tests. Permutation Tests, Rank Tests. Interval Estimation, Confidence Interval, Confidence, Confidence Region. Point Estimation, Bias, Mean Square Error. Assignments are returned using LaTeX and consitute 20% of the final grade.
Latent variable models I (SÁL138F)
The course covers models used to work with the underlying variables in psychological measurements will be introduced. In the first course (Models for underlying variables I) we will work with confirmatory factor models and structural equation models (also known as path models). We will cover the assumptions of the models, how to work with them, and interpretation of results. Methods to work with different types of data will be discussed. In the second course (Models for underlying variables II) we will start by introducing methods for categorical variables and then move to the closely related item response models. Primary focus will be on models for binary data but the most common models for categorical data will be introduced. In the second part of the course, we will move on to models for longitudinal data that use underlying variables: latent growth models, cross-lagged product models, and models for intensive longitudinal methods (also known as Daily-diary data, Ambulatory assessment, or Ecological-momentary data). Emphasis will be on practical training in analyzing data with models through projects, as well as the theoretical basis of models.
Biometry (LÍF127F)
Numerical methods are an essential part of biology and are applied to design of experiments and observations, description of result and their analysis. Sudents learn these methods by working on biological data and to interpretate its results. Main method include the maximum likelihood estimation, linear models, regression and analysis of variance and generalized linear models. Multivariate analysis. Bootstrap and permutation analysis. The analysis will done using R. The students will obtain an extensive exercise in applyin R on various biological datasets. Analysis of own data or an extensive dataset, presented in a report and a lecture.
Assessment: Written examen 50%, assignments, report and lecture (50%).
Construction of self report scales (SÁL139F)
This course is designed to introduce students to the practice of psychological scale development and testing. Classical test theory is introduced with an emphasis on understanding statistical concepts related to scale construction. The main focus of the course is on practical training in scale development and the controversial issues related to developing a psychological scale from scratch.
Human Genetics (LÍF513M)
Lectures: Mendelian genetics, organization of the human genome, structure of chromosomes, chromosomal changes and syndromes, gene mapping via association and whole genome sequencing methods, genetic analysis, genetic screening, genetics of simple and complex traits, genes and environment, cancer genetics, gene therapy, human and primate evolution, ethical issues concerning human genetics, informed consent and private information. Students are expected to have prior knowledge of the principles genetics.
Practical: Analyses of genetic data, study of chromosomal labelling, analyses of genetic associations and transcriptomes.
Time Series Analysis (IÐN113F)
ARMAX and other similar time series models. Non-stationary time series. Correlation and spectral analysis. Parameter estimation, parametric and non-parametric approaches, Least Squares and Maximum Likelihood. Model validation methods. Models with time dependent parameters. Numerical methods for minimization. Outlier detection and interpolation. Introduction to nonlinear time series models. Discrete state space models. Discrete state space models. Extensive use of MATLAB, especially the System Identification Toolbox.
Theory of linear models (STÆ310M)
Simple and multiple linear regression, analysis of variance and covariance, inference, variances and covariances of estimators, influence and diagnostic analyses using residual and influence measures, simultaneous inference. General linear models as projections with ANOVA as special case, simultaneous inference of estimable functions. R is used in assignments. Solutions to assignments are returned in LaTeX and PDF format.
In addition selected topics will be visited, e.g. generalized linear models (GLMs), nonlinear regression and/or random/mixed effects models and/or bootstrap methods etc.
Students will present solutions to individually assigned
projects/exercises, each of which is handed in earlier through a web-page.
This course is taught in semesters of even-numbered years.
Computing and Calculus for Applied Statistics (STÆ012F)
Univariate calculus (basic algebra, functi ons, polynomials, logarithms and exponenti al functi ons, conti nuity and limits, diff erenti ati on, local extrema andintegrati on).
Linear algebra (vectors, matrices, linear projecti ons with matrices, matrix inverses and determinants).
Programming in R (arithmeti c, functi ons and organizing R code).
Multi variate calculus (Jacobian, Hessian and double integrals).
The approach will be to address each mathemati cs topic using a mix of (a) basic theory (in the form of concepts rather than proofs), (b) computerprogramming using R to visualize the theory, and (c) examples exclusively from stati stics. Formal lectures are not planned, but students will be able toseek assistance with their weekly assignments.
The goal of the course is to cover the calculus, linear algebra and computer programming concepts most commonly needed in stati sti cs. A student who has completed this course should have the mathematical basis for statistics courses currently taught at the MSc level by the mathematics department(and thus also for all stati sti cs courses taught by other departments).
Students will author examples and multiple-choice questi ons, and their submissions will be graded by their peers.
The material is openly available at https://open-educati on-hub.github.io/ccas/.
Epidemiologic Methods (Epidemiology II) (LÝÐ085F)
The aim of the course is to increase students‘ understanding of advanced methods in epidemiology and to enhance students‘ ability to interpret results and assess the quality of scientific research in epidemiology.
The course will cover positive and negative confounding, matching, propensity score, effect modification and interaction, instrumental variables, causal diagrams, and missing data. Scientific articles in epidemiology will be studied and discussed.
Random Effects Models (STÆ004F)
The focus of this course is on Bayesian latent Gaussian models (BLGMs) which are a class of Bayesian hierarchical models and applications of these models. The main topics are three types of BLGMs: (i) Bayesian Gaussian—Gaussian models, (ii) BLGMs with a univariate link function, and (iii) BLGMs with a multivariate link function, as well as prior densities for BLGMs and posterior computation for BLGMs. In the first part of the course, the basics of these models is covered and homework assignments will be given on these topics. In the second part of the course, the focus is on a project, in which data are analyzed using BLGMs. Each student can contribute data that she or he wishes to analyze. The material in the course is based on a theoretical background. However, the focus on data analysis is strong, and computation and programming play a large role in the course. Thus, the course will be useful to students in their future projects involving data analysis.
Linear regression models, the multiple normal distribution, hierarchical models, fixed and random effect models, restricted maximum likelihood estimation, best linear unbiased estimators, Bayesian inference, statistical decision theory, Markov chains, Monte Carlo integration, importance sampling, Markov chain Monte Carlo, Gibbs sampling, the Metropolis-Hastings algorithm.
Stochastic Processes (STÆ415M)
Introduction to stochastic processes with main emphasis on Markov chains.
Subject matter: Hitting time, classification of states, irreducibility, period, recurrence (positive and null), transience, regeneration, coupling, stationarity, time-reversibility, coupling from the past, branching processes, queues, martingales, Brownian motion.
Trauma and its impact on health (LÝÐ0A0F)
This course describes trauma in childhood and adulthood, including violence, accidents, disasters and life-threatening illness and their association with mental and physical health. Emphasis will be placed on introducing the scientific foundation of the trauma field and understanding scientific articles in this area. The main topics of the course include:
- Prevalence of traumatic events and acute stress reactions.
- Mental health problems following trauma, such as posttraumatic stress disorder, anxiety, depression, sleep disturbances, substance abuse and prolonged grief.
- The disease burden of trauma, due to e.g., cardiovascular diseases, cancers, autoimmune diseases, and suicide.
- The influence of environmental and genetic factors in the development of psychological and physical diseases following trauma.
- Factors that promote recovery post-trauma and reduce the risk of long-term health problems.
- Evidence-based treatment options for PTSD.
The course is intended for students who want to increase their scientific knowledge of the relationship between trauma and health. It is only intended for postgraduate students. The course consists of lectures by the main supervisor and selected guest speakers who are experts in the field of trauma. Emphasis will be placed on discussions and active participation of students.
Applied data analysis (MAS202M)
The course focuses on statistical analysis using the R environment. It is assumed that students have basic knowledge of statistics and the statistical software R. Students will learn to apply a broad range of statistical methods in R (such as classification methods, resampling methods, linear model selection and tree-based methods). The course on 12 weeks and will be on "flipped" form. This means that no lectures will be given but students will read some material and watch videos before attending classes. Students will then work on assignments during the classes.
Applied multivariable regression and data analysis (NÆR506M)
The aim of this course is to enable student to conduct their own data analyses. This includes familiarizing them with practical aspects of data cleaning/processing and statistical methods used within nutritional epidemiology.
Short lectures will be given covering selected subjects followed by practical assignments. Assignments will contribute 100% to the final grade.
Some experience with SPSS, SAS or related softwere in addition to having taken basic course in statistics is desierable, but not required.
Social science statistics: Regression analysis (FMÞ501M)
This is a comprehensive course in multiple-regression analysis. The goal of the course is that students develop enough conceptual understanding and practical knowledge to use this method on their own. The lectures cover various regression analysis techniques commonly used in quantitative social research, including control variables, the use of nominal variables, linear and nonlinear models, techniques that test for mediation and statistical interaction effects, and so on. We discuss the assumptions of regression analysis and learn techniques to detect and deal with violations of assumptions. In addition, logistic regression will be introduced, which is a method for a dichotomous dependent variable. We also review many of the basic concepts involved in statistical inference and significance testing. Students get plenty of hands-on experience with data analysis. The instructor hands out survey data that students use to practice the techniques covered in class. The statistical package SPSS will be used.
Genomics and bioinformatics (LÍF659M)
Genomics and bioinformatics are intertwinned in many ways. Technological advances enabled the sequencing of for instance genomes, transcriptomes and proteomes. Complete genome sequences of thousands of organisms enables study of this flood of information for gaining knowledge and deeper understanding of biological phenomena. Comparative studies, in one way or another, building on Darwininan thought provide the theoretical underpinnings for analyzing this information and it applications. Characters and features conserved among organisms are based in conserved parts of genomes and conversely, new and unique phenotypes are affected by variable parts of genomes. This applies equally to animals, plants and microbes, and cells, enzymatic and developmental systems.
The course centers on the theoretical and practical aspects of comparative analysis, about analyses of genomes, metagenomes and transcriptomes to study biological, medical and applied questions. The lectures cover structure and sequencing of genomes, transcriptomes and proteomes, molecular evolution, different types of bioinformatic data, shell scripts, intro to R and Python scripting and applications. The practicals include, retrieval of data from databases, blast and alignment, assembly and annotation, comparison of genomes, population data analyses. Students will work with databases, such as Flybase, Genebank and ENSEMBL. Data will be retrived with Biomart and Bioconductor, and data quality discussed. Algorithms for search tools and alignments, read counts and comparisons of groups and treatments. Also elements of python scripting, open linux software, installation of linux programs, analyses of data from RNA-seq, RADseq and genome sequencing.
Students are required to turn in a few small and one big group project and present the large project with a lecture. In discussion session primary literature will be presented.
Molecular Genetics (LÍF644M)
Lectures: The molecular basis of life (chemical bonds, biological molecules, structure of DNA, RNA and proteins). Genomes and the flow of biological information. Chromosome structure and function, chromatin and nucleosomes. The cell cycle, DNA replication. Chromosome segregaition, Transcription. Regulation of transcription. RNA processing. Translation. Regulation of translation. Regulatory RNAs. Protein modification and targeting. DNA damage, checkpoints and DNA repair mechanisms. Repair of DNA double-strand breaks and homologous recombination. Mobile DNA elements. Tools and techniques in molecular Biology icluding Model organisms.
Seminar: Students present and discuss selected research papers and hand in a short essay.
Laboratory work: Work on molecular genetics project relevant to current research. Basic methods such as gene cloning, gene transfer and expression, PCR, sequencing, DNA isolation and restriction analysis, electrophoresis of DNA and proteins will be used.
Exam: Laboratory 10%, seminar 15%, written final exam 75%.
Introduction to Measure-Theoretic Probability (STÆ418M)
Probability based on measure-theory.
Subject matter: Probability, extension theorems, independence, expectation. The Borel-Cantelli theorem and the Kolmogorov 0-1 law. Inequalities and the weak and strong laws of large numbers. Convergence pointwise, in probability, with probability one, in distribtution, and in total variation. Coupling methods. The central limit theorem. Conditional probability and expectation.
Genomics and bioinformatics (LÍF659M)
Genomics and bioinformatics are intertwinned in many ways. Technological advances enabled the sequencing of for instance genomes, transcriptomes and proteomes. Complete genome sequences of thousands of organisms enables study of this flood of information for gaining knowledge and deeper understanding of biological phenomena. Comparative studies, in one way or another, building on Darwininan thought provide the theoretical underpinnings for analyzing this information and it applications. Characters and features conserved among organisms are based in conserved parts of genomes and conversely, new and unique phenotypes are affected by variable parts of genomes. This applies equally to animals, plants and microbes, and cells, enzymatic and developmental systems.
The course centers on the theoretical and practical aspects of comparative analysis, about analyses of genomes, metagenomes and transcriptomes to study biological, medical and applied questions. The lectures cover structure and sequencing of genomes, transcriptomes and proteomes, molecular evolution, different types of bioinformatic data, shell scripts, intro to R and Python scripting and applications. The practicals include, retrieval of data from databases, blast and alignment, assembly and annotation, comparison of genomes, population data analyses. Students will work with databases, such as Flybase, Genebank and ENSEMBL. Data will be retrived with Biomart and Bioconductor, and data quality discussed. Algorithms for search tools and alignments, read counts and comparisons of groups and treatments. Also elements of python scripting, open linux software, installation of linux programs, analyses of data from RNA-seq, RADseq and genome sequencing.
Students are required to turn in a few small and one big group project and present the large project with a lecture. In discussion session primary literature will be presented.
Algorithms in Bioinformatics (TÖL604M)
This course will cover the algorithmic aspects of bioinformatic. We will start with an introduction to genomics and algorithms for students new to the field. The course is divided into modules, each module covers a single problem in bioinformatics that will be motivated based on current research. The module will then cover algorithms that solve the problem and variations on the problem. The problems covered are motif finding, edit distance in strings, sequence alignment, clustering, sequencing and assembly and finally computational methods for high throughput sequencing (HTS).
- Fall
- STÆ312MApplied Linear Statistical ModelsMandatory (required) course6A mandatory (required) course for the programme6 ECTS, creditsCourse Description
The course focuses on simple and multiple linear regression as well as analysis of variance (ANOVA), analysis of covariance (ANCOVA) and binomial regression. The course is a natural continuation of a typical introductory course in statistics taught in various departments of the university.
We will discuss methods for estimating parameters in linear models, how to construct confidence intervals and test hypotheses for the parameters, which assumptions need to hold for applying the models and what to do when they are not met.
Students will work on projects using the statistical software R.
Face-to-face learningPrerequisitesLÝÐ301FBiostatistics II (Clinical Prediction Models )Mandatory (required) course6A mandatory (required) course for the programme6 ECTS, creditsCourse DescriptionThis course is a continuation of Biostatistics I and constitutes a practical guide to statistical analyses of student's own research projects. The course covers the following topics. Estimation of relative risk/odds ratios and adjusted estimation of relative risk/odds ratios, correlation and simple linear regression, multiple linear regression and logistic regression. The course is based on lectures and practical sessions using R for statistical analyses.
Face-to-face learningPrerequisitesCourse taught first half of the semesterLÝÐ108FOrientation seminar: public health, epidemiology and biostatisticsMandatory (required) course1A mandatory (required) course for the programme1 ECTS, creditsCourse DescriptionThis is a preparatory course for students in these interdisciplinary and research based studies. The course covers various practical issues, methods, study planning, refernce search, and scientific literacy e.g.. Students also aquire basic training in the statistical program R.
Face-to-face learningPrerequisitesAttendance required in classLÝÐ107FEpidemiology - a quantitative methodologyMandatory (required) course6A mandatory (required) course for the programme6 ECTS, creditsCourse DescriptionThe course is an introduction to epidemiological research methods and causal inference. An overview is provided on measure of disease occurrence, measures of outcome (relative risks), and study design (experiments, intervention studies, cohort studies and case-control studies). Emphasis is on systematic errors and on methods to avoid such errors in planning (study design) and in data analyses. Students get training in reviewing epidemiological studies.
Face-to-face learningPrerequisitesMAS102MR ProgrammingMandatory (required) course3A mandatory (required) course for the programme3 ECTS, creditsCourse DescriptionStudents will perform traditional statistical analysis on real data sets. Special focus will be on regression methods, including multiple regression analysis. Students will apply sophisticated methods of graphical representation and automatic reporting. Students will hand in a projects where they apply the above mentioned methods on real datasets in order to answer research questions
Face-to-face learningPrerequisites- Spring 2
LÝÐ079FBiostatistics III (Survival analysis)Mandatory (required) course6A mandatory (required) course for the programme6 ECTS, creditsCourse DescriptionThe course covers methods for analysis of cohort studies using methods for time to event or survival analysis. It is based on the course Biostat III – Survival analysis for epidemiologists in R at the Karolinska Institutet: See (https://biostat3.net/index.html): "Topics covered include methods for estimating patient survival (life table and Kaplan-Meier methods), comparing survival between patient subgroups (log-rank test), and modelling survival (primarily Poisson regression, Cox proportional hazards model and flexible parametric models). The course addresses the concept of 'time' as a potential confounder or effect modifier and approaches to defining 'time' (e.g., time since entry, attained age, calendar time). The course will emphasise the basic concepts of statistical modelling in epidemiology, such as controlling for confounding and assessing effect modification."
Face-to-face learningPrerequisitesNot taught this semesterMAS201MStatistical ConsultingMandatory (required) course6A mandatory (required) course for the programme6 ECTS, creditsCourse DescriptionParticipants in the course will obtain training in practical statistics as used when providing general statistical counselling. The participants will be introduced to actual statistical projects by assisting students in various departments within the university. The participants will report on the projects in class, discuss options for solving the projects and subsequently assist the students with analyses using R and interpretation of results.
Face-to-face learningPrerequisitesLÝÐ202FThe Scientific Process: Ethics, Communication and PracticalitiesMandatory (required) course6A mandatory (required) course for the programme6 ECTS, creditsCourse DescriptionThe course constitutes a practical guide to the preparation of a health-related research study. Modules include: reference search and handling, development of hypotheses, creation of a systematic critical review within chosen field of research, development and presentation of research proposals.
The course is for graduate students who have chosen a field/research question for their dissertation project.
Students in MPH Public Health Sciences and MS Epidemiology and Biostatistics must pass the course before starting work on their final thesis.
Face-to-face learningPrerequisitesCourse taught second half of the semester- Whole year courses
- LÝÐ098FResearch Training in Public Health SciencesElective course8Free elective course within the programme8 ECTS, creditsCourse Description
The aim of the course is for students to gain training in methods and insight into the implementation of a specific research project in public health. Students get to know the theoretical background of the research they are working on and are trained to participate in a defined part of it, but the topics depend on the needs of the research project that is assigned. An example would be e.g. participation in the preparation of a research project and various work related to data collection and data processing (cleaning and/or analysis). Students will also get to know the different aspects of the research project under the guidance of the scientific staff leading the research and in collaboration with the research team.
Students attend regular meetings with the researchers and supervisors of the course. The research training is planned specifically for each student with regard to the content and progress of the research, the supervisor and the student's background. Study space is limited by the research projects that are ongoing at the Center for Public Health Sciences at any given time, and students apply for registration for the course at the program office.
Face-to-face learningPrerequisites- Fall
LÝÐ060LThesis in Epidemiology and BiostatisticsMandatory (required) course30A mandatory (required) course for the programme30 ECTS, creditsCourse DescriptionThe MS program in Epidemiology and Biostatistics is a 120 credit post-graduate and cross-disciplinary program, including a 30 or 60 credit research thesis. Projects that have been/could be submitted for publication can be awarded 60 credits. During the first semester students develop their research questions and choose a thesis advisor. A complete research proposal is presented at the end of the second semester. Departmental registration is determined by the students' area of interest and host faculty of their main advisor.
The objective of the MS thesis is to provide training in constructing, organising, developing and conducting a research or developmental project in the public health sciences and an understanding of the technical limits, regulations, ethics and laws that must be observed in such work. Students should be able to define and present research questions and propose original hypotheses.
Self-studyPrerequisitesPart of the total project/thesis credits- Spring 2
LÝÐ060LThesis in Epidemiology and BiostatisticsMandatory (required) course30A mandatory (required) course for the programme30 ECTS, creditsCourse DescriptionThe MS program in Epidemiology and Biostatistics is a 120 credit post-graduate and cross-disciplinary program, including a 30 or 60 credit research thesis. Projects that have been/could be submitted for publication can be awarded 60 credits. During the first semester students develop their research questions and choose a thesis advisor. A complete research proposal is presented at the end of the second semester. Departmental registration is determined by the students' area of interest and host faculty of their main advisor.
The objective of the MS thesis is to provide training in constructing, organising, developing and conducting a research or developmental project in the public health sciences and an understanding of the technical limits, regulations, ethics and laws that must be observed in such work. Students should be able to define and present research questions and propose original hypotheses.
Self-studyPrerequisitesPart of the total project/thesis credits- Fall
- LÝÐ101FPublic Health: Science, Politics, PreventionRestricted elective course6Restricted elective course, conditions apply6 ECTS, creditsCourse Description
The course provides an overview of definitions, history, aims, legislation, methods and ethical considerations in public health and public health sciences. The course lays emphasis on global public health as well as on the Icelandic health care system, its administration and funding in comparison with health care systems in other nations. An overview is provided on Icelandic and international databases on health and disease and possibilities for their utilization in research and policy making for health promotion. In addition, current public health issues at each time are emphasized.
Face-to-face learningPrerequisitesAttendance required in classCourse taught first half of the semesterSTÆ529MBayesian Data AnalysisRestricted elective course8Restricted elective course, conditions apply8 ECTS, creditsCourse DescriptionGoal: To train students in applying methods of Bayesian statistics for analysis of data. Topics: Theory of Bayesian inference, prior distributions, data distributions and posterior distributions. Bayesian inference for parameters of univariate and multivariate distributions: binomial; normal; Poisson; exponential; multivariate normal; multinomial. Model checking and model comparison: Bayesian p-values; deviance information criterion (DIC). Bayesian computation: Markov chain Monte Carlo (MCMC) methods; the Gibbs sampler; the Metropolis-Hastings algorithm; convergence diagnostistics. Linear models: normal linear models; hierarchical linear models; generalized linear models. Emphasis on data analysis using software, e.g. Matlab and R.
Face-to-face learningThe course is taught if the specified conditions are metPrerequisitesLÝÐ0A1FMixed Linear ModelsRestricted elective course6Restricted elective course, conditions apply6 ECTS, creditsCourse DescriptionThe course is about the theory and application of random effects, or linear mixed models, and related models for correlated response variables. The course will cover methods for continuous and approximately normally distributed variables. A statistical model for such data has to describe both the expected value and the covariance between observations. The theory extends the theory of general linear models. Special software is needed for such an analysis and the necessary packages are provided in R, STATA, and SAS. The application will be based on R but other programs will be introduced for comparison.
The course will be taught between beginning of September and end of November, meeting once a week. The teaching will be in a flipped class manner. All the material (both notes and lectures) are online (see below) and it is expected that students will have viewed the material before class. In class the theory will be discussed and then students are expected to work on the application on their own computer.Face-to-face learningPrerequisitesLÝÐ097FTopics in Epidemiology (Epidemiology III)Restricted elective course6Restricted elective course, conditions apply6 ECTS, creditsCourse DescriptionThe aim of the course is to increase students' understanding of different areas within epidemiology, provide an introduction to area-specific methods, and to enhance students' ability to interpret results and assess the quality of scientific research in epidemiology.
The course will cover 4-6 specific areas or topics within epidemiology. Examples include perinatal, nutritional, pharmacological, and infectious disease epidemiology; featured topics may vary from year to year.
Face-to-face learningPrerequisitesSTÆ313MTheoretical StatisticsRestricted elective course10Restricted elective course, conditions apply10 ECTS, creditsCourse DescriptionLikelihood, Sufficient Statistic, Sufficiency Principle, Nuisance Parameter, Conditioning Principle, Invariance Principle, Likelihood Theory. Hypothesis Testing, Simple and Composite Hypothesis, The Neyman-Pearson Lemma, Power, UMP-Test, Invariant Tests. Permutation Tests, Rank Tests. Interval Estimation, Confidence Interval, Confidence, Confidence Region. Point Estimation, Bias, Mean Square Error. Assignments are returned using LaTeX and consitute 20% of the final grade.
Face-to-face learningOnline learningThe course is taught if the specified conditions are metPrerequisitesSÁL138FLatent variable models IRestricted elective course8Restricted elective course, conditions apply8 ECTS, creditsCourse DescriptionThe course covers models used to work with the underlying variables in psychological measurements will be introduced. In the first course (Models for underlying variables I) we will work with confirmatory factor models and structural equation models (also known as path models). We will cover the assumptions of the models, how to work with them, and interpretation of results. Methods to work with different types of data will be discussed. In the second course (Models for underlying variables II) we will start by introducing methods for categorical variables and then move to the closely related item response models. Primary focus will be on models for binary data but the most common models for categorical data will be introduced. In the second part of the course, we will move on to models for longitudinal data that use underlying variables: latent growth models, cross-lagged product models, and models for intensive longitudinal methods (also known as Daily-diary data, Ambulatory assessment, or Ecological-momentary data). Emphasis will be on practical training in analyzing data with models through projects, as well as the theoretical basis of models.
Face-to-face learningPrerequisitesNot taught this semesterLÍF127FBiometryRestricted elective course8Restricted elective course, conditions apply8 ECTS, creditsCourse DescriptionNumerical methods are an essential part of biology and are applied to design of experiments and observations, description of result and their analysis. Sudents learn these methods by working on biological data and to interpretate its results. Main method include the maximum likelihood estimation, linear models, regression and analysis of variance and generalized linear models. Multivariate analysis. Bootstrap and permutation analysis. The analysis will done using R. The students will obtain an extensive exercise in applyin R on various biological datasets. Analysis of own data or an extensive dataset, presented in a report and a lecture.
Assessment: Written examen 50%, assignments, report and lecture (50%).
Face-to-face learningPrerequisitesCourse taught in period ISÁL139FConstruction of self report scalesElective course8Free elective course within the programme8 ECTS, creditsCourse DescriptionThis course is designed to introduce students to the practice of psychological scale development and testing. Classical test theory is introduced with an emphasis on understanding statistical concepts related to scale construction. The main focus of the course is on practical training in scale development and the controversial issues related to developing a psychological scale from scratch.
Face-to-face learningPrerequisitesNot taught this semesterLÍF513MHuman GeneticsElective course6Free elective course within the programme6 ECTS, creditsCourse DescriptionLectures: Mendelian genetics, organization of the human genome, structure of chromosomes, chromosomal changes and syndromes, gene mapping via association and whole genome sequencing methods, genetic analysis, genetic screening, genetics of simple and complex traits, genes and environment, cancer genetics, gene therapy, human and primate evolution, ethical issues concerning human genetics, informed consent and private information. Students are expected to have prior knowledge of the principles genetics.
Practical: Analyses of genetic data, study of chromosomal labelling, analyses of genetic associations and transcriptomes.
Face-to-face learningPrerequisitesAttendance required in classIÐN113FTime Series AnalysisElective course7,5Free elective course within the programme7,5 ECTS, creditsCourse DescriptionARMAX and other similar time series models. Non-stationary time series. Correlation and spectral analysis. Parameter estimation, parametric and non-parametric approaches, Least Squares and Maximum Likelihood. Model validation methods. Models with time dependent parameters. Numerical methods for minimization. Outlier detection and interpolation. Introduction to nonlinear time series models. Discrete state space models. Discrete state space models. Extensive use of MATLAB, especially the System Identification Toolbox.
Distance learningSelf-studyPrerequisitesNot taught this semesterSTÆ310MTheory of linear modelsElective course6Free elective course within the programme6 ECTS, creditsCourse DescriptionSimple and multiple linear regression, analysis of variance and covariance, inference, variances and covariances of estimators, influence and diagnostic analyses using residual and influence measures, simultaneous inference. General linear models as projections with ANOVA as special case, simultaneous inference of estimable functions. R is used in assignments. Solutions to assignments are returned in LaTeX and PDF format.
In addition selected topics will be visited, e.g. generalized linear models (GLMs), nonlinear regression and/or random/mixed effects models and/or bootstrap methods etc.
Students will present solutions to individually assigned
projects/exercises, each of which is handed in earlier through a web-page.This course is taught in semesters of even-numbered years.
Face-to-face learningOnline learningThe course is taught if the specified conditions are metPrerequisitesSTÆ012FComputing and Calculus for Applied StatisticsElective course8Free elective course within the programme8 ECTS, creditsCourse DescriptionUnivariate calculus (basic algebra, functi ons, polynomials, logarithms and exponenti al functi ons, conti nuity and limits, diff erenti ati on, local extrema andintegrati on).
Linear algebra (vectors, matrices, linear projecti ons with matrices, matrix inverses and determinants).
Programming in R (arithmeti c, functi ons and organizing R code).
Multi variate calculus (Jacobian, Hessian and double integrals).
The approach will be to address each mathemati cs topic using a mix of (a) basic theory (in the form of concepts rather than proofs), (b) computerprogramming using R to visualize the theory, and (c) examples exclusively from stati stics. Formal lectures are not planned, but students will be able toseek assistance with their weekly assignments.
The goal of the course is to cover the calculus, linear algebra and computer programming concepts most commonly needed in stati sti cs. A student who has completed this course should have the mathematical basis for statistics courses currently taught at the MSc level by the mathematics department(and thus also for all stati sti cs courses taught by other departments).Students will author examples and multiple-choice questi ons, and their submissions will be graded by their peers.
The material is openly available at https://open-educati on-hub.github.io/ccas/.
Face-to-face learningPrerequisites- Spring 2
LÝÐ085FEpidemiologic Methods (Epidemiology II)Restricted elective course6Restricted elective course, conditions apply6 ECTS, creditsCourse DescriptionThe aim of the course is to increase students‘ understanding of advanced methods in epidemiology and to enhance students‘ ability to interpret results and assess the quality of scientific research in epidemiology.
The course will cover positive and negative confounding, matching, propensity score, effect modification and interaction, instrumental variables, causal diagrams, and missing data. Scientific articles in epidemiology will be studied and discussed.
Face-to-face learningPrerequisitesSTÆ004FRandom Effects ModelsRestricted elective course8Restricted elective course, conditions apply8 ECTS, creditsCourse DescriptionThe focus of this course is on Bayesian latent Gaussian models (BLGMs) which are a class of Bayesian hierarchical models and applications of these models. The main topics are three types of BLGMs: (i) Bayesian Gaussian—Gaussian models, (ii) BLGMs with a univariate link function, and (iii) BLGMs with a multivariate link function, as well as prior densities for BLGMs and posterior computation for BLGMs. In the first part of the course, the basics of these models is covered and homework assignments will be given on these topics. In the second part of the course, the focus is on a project, in which data are analyzed using BLGMs. Each student can contribute data that she or he wishes to analyze. The material in the course is based on a theoretical background. However, the focus on data analysis is strong, and computation and programming play a large role in the course. Thus, the course will be useful to students in their future projects involving data analysis.
Linear regression models, the multiple normal distribution, hierarchical models, fixed and random effect models, restricted maximum likelihood estimation, best linear unbiased estimators, Bayesian inference, statistical decision theory, Markov chains, Monte Carlo integration, importance sampling, Markov chain Monte Carlo, Gibbs sampling, the Metropolis-Hastings algorithm.
Face-to-face learningPrerequisitesNot taught this semesterSTÆ415MStochastic ProcessesElective course10Free elective course within the programme10 ECTS, creditsCourse DescriptionIntroduction to stochastic processes with main emphasis on Markov chains.
Subject matter: Hitting time, classification of states, irreducibility, period, recurrence (positive and null), transience, regeneration, coupling, stationarity, time-reversibility, coupling from the past, branching processes, queues, martingales, Brownian motion.Face-to-face learningThe course is taught if the specified conditions are metPrerequisitesLÝÐ0A0FTrauma and its impact on healthElective course6Free elective course within the programme6 ECTS, creditsCourse DescriptionThis course describes trauma in childhood and adulthood, including violence, accidents, disasters and life-threatening illness and their association with mental and physical health. Emphasis will be placed on introducing the scientific foundation of the trauma field and understanding scientific articles in this area. The main topics of the course include:
- Prevalence of traumatic events and acute stress reactions.
- Mental health problems following trauma, such as posttraumatic stress disorder, anxiety, depression, sleep disturbances, substance abuse and prolonged grief.
- The disease burden of trauma, due to e.g., cardiovascular diseases, cancers, autoimmune diseases, and suicide.
- The influence of environmental and genetic factors in the development of psychological and physical diseases following trauma.
- Factors that promote recovery post-trauma and reduce the risk of long-term health problems.
- Evidence-based treatment options for PTSD.
The course is intended for students who want to increase their scientific knowledge of the relationship between trauma and health. It is only intended for postgraduate students. The course consists of lectures by the main supervisor and selected guest speakers who are experts in the field of trauma. Emphasis will be placed on discussions and active participation of students.
Distance learningPrerequisitesAttendance required in classCourse taught second half of the semesterMAS202MApplied data analysisElective course6Free elective course within the programme6 ECTS, creditsCourse DescriptionThe course focuses on statistical analysis using the R environment. It is assumed that students have basic knowledge of statistics and the statistical software R. Students will learn to apply a broad range of statistical methods in R (such as classification methods, resampling methods, linear model selection and tree-based methods). The course on 12 weeks and will be on "flipped" form. This means that no lectures will be given but students will read some material and watch videos before attending classes. Students will then work on assignments during the classes.
Face-to-face learningPrerequisitesNÆR506MApplied multivariable regression and data analysisElective course6Free elective course within the programme6 ECTS, creditsCourse DescriptionThe aim of this course is to enable student to conduct their own data analyses. This includes familiarizing them with practical aspects of data cleaning/processing and statistical methods used within nutritional epidemiology.
Short lectures will be given covering selected subjects followed by practical assignments. Assignments will contribute 100% to the final grade.
Some experience with SPSS, SAS or related softwere in addition to having taken basic course in statistics is desierable, but not required.
Face-to-face learningPrerequisitesFMÞ501MSocial science statistics: Regression analysisElective course10Free elective course within the programme10 ECTS, creditsCourse DescriptionThis is a comprehensive course in multiple-regression analysis. The goal of the course is that students develop enough conceptual understanding and practical knowledge to use this method on their own. The lectures cover various regression analysis techniques commonly used in quantitative social research, including control variables, the use of nominal variables, linear and nonlinear models, techniques that test for mediation and statistical interaction effects, and so on. We discuss the assumptions of regression analysis and learn techniques to detect and deal with violations of assumptions. In addition, logistic regression will be introduced, which is a method for a dichotomous dependent variable. We also review many of the basic concepts involved in statistical inference and significance testing. Students get plenty of hands-on experience with data analysis. The instructor hands out survey data that students use to practice the techniques covered in class. The statistical package SPSS will be used.
Face-to-face learningPrerequisitesLÍF659MGenomics and bioinformaticsElective course6Free elective course within the programme6 ECTS, creditsCourse DescriptionGenomics and bioinformatics are intertwinned in many ways. Technological advances enabled the sequencing of for instance genomes, transcriptomes and proteomes. Complete genome sequences of thousands of organisms enables study of this flood of information for gaining knowledge and deeper understanding of biological phenomena. Comparative studies, in one way or another, building on Darwininan thought provide the theoretical underpinnings for analyzing this information and it applications. Characters and features conserved among organisms are based in conserved parts of genomes and conversely, new and unique phenotypes are affected by variable parts of genomes. This applies equally to animals, plants and microbes, and cells, enzymatic and developmental systems.
The course centers on the theoretical and practical aspects of comparative analysis, about analyses of genomes, metagenomes and transcriptomes to study biological, medical and applied questions. The lectures cover structure and sequencing of genomes, transcriptomes and proteomes, molecular evolution, different types of bioinformatic data, shell scripts, intro to R and Python scripting and applications. The practicals include, retrieval of data from databases, blast and alignment, assembly and annotation, comparison of genomes, population data analyses. Students will work with databases, such as Flybase, Genebank and ENSEMBL. Data will be retrived with Biomart and Bioconductor, and data quality discussed. Algorithms for search tools and alignments, read counts and comparisons of groups and treatments. Also elements of python scripting, open linux software, installation of linux programs, analyses of data from RNA-seq, RADseq and genome sequencing.
Students are required to turn in a few small and one big group project and present the large project with a lecture. In discussion session primary literature will be presented.Face-to-face learningDistance learningThe course is taught if the specified conditions are metPrerequisitesCourse DescriptionLectures: The molecular basis of life (chemical bonds, biological molecules, structure of DNA, RNA and proteins). Genomes and the flow of biological information. Chromosome structure and function, chromatin and nucleosomes. The cell cycle, DNA replication. Chromosome segregaition, Transcription. Regulation of transcription. RNA processing. Translation. Regulation of translation. Regulatory RNAs. Protein modification and targeting. DNA damage, checkpoints and DNA repair mechanisms. Repair of DNA double-strand breaks and homologous recombination. Mobile DNA elements. Tools and techniques in molecular Biology icluding Model organisms.
Seminar: Students present and discuss selected research papers and hand in a short essay.
Laboratory work: Work on molecular genetics project relevant to current research. Basic methods such as gene cloning, gene transfer and expression, PCR, sequencing, DNA isolation and restriction analysis, electrophoresis of DNA and proteins will be used.
Exam: Laboratory 10%, seminar 15%, written final exam 75%.
Face-to-face learningPrerequisitesSTÆ418MIntroduction to Measure-Theoretic ProbabilityElective course10Free elective course within the programme10 ECTS, creditsCourse DescriptionProbability based on measure-theory.
Subject matter: Probability, extension theorems, independence, expectation. The Borel-Cantelli theorem and the Kolmogorov 0-1 law. Inequalities and the weak and strong laws of large numbers. Convergence pointwise, in probability, with probability one, in distribtution, and in total variation. Coupling methods. The central limit theorem. Conditional probability and expectation.
Face-to-face learningThe course is taught if the specified conditions are metPrerequisitesLÍF659MGenomics and bioinformaticsElective course6Free elective course within the programme6 ECTS, creditsCourse DescriptionGenomics and bioinformatics are intertwinned in many ways. Technological advances enabled the sequencing of for instance genomes, transcriptomes and proteomes. Complete genome sequences of thousands of organisms enables study of this flood of information for gaining knowledge and deeper understanding of biological phenomena. Comparative studies, in one way or another, building on Darwininan thought provide the theoretical underpinnings for analyzing this information and it applications. Characters and features conserved among organisms are based in conserved parts of genomes and conversely, new and unique phenotypes are affected by variable parts of genomes. This applies equally to animals, plants and microbes, and cells, enzymatic and developmental systems.
The course centers on the theoretical and practical aspects of comparative analysis, about analyses of genomes, metagenomes and transcriptomes to study biological, medical and applied questions. The lectures cover structure and sequencing of genomes, transcriptomes and proteomes, molecular evolution, different types of bioinformatic data, shell scripts, intro to R and Python scripting and applications. The practicals include, retrieval of data from databases, blast and alignment, assembly and annotation, comparison of genomes, population data analyses. Students will work with databases, such as Flybase, Genebank and ENSEMBL. Data will be retrived with Biomart and Bioconductor, and data quality discussed. Algorithms for search tools and alignments, read counts and comparisons of groups and treatments. Also elements of python scripting, open linux software, installation of linux programs, analyses of data from RNA-seq, RADseq and genome sequencing.
Students are required to turn in a few small and one big group project and present the large project with a lecture. In discussion session primary literature will be presented.Face-to-face learningDistance learningThe course is taught if the specified conditions are metPrerequisitesTÖL604MAlgorithms in BioinformaticsElective course6Free elective course within the programme6 ECTS, creditsCourse DescriptionThis course will cover the algorithmic aspects of bioinformatic. We will start with an introduction to genomics and algorithms for students new to the field. The course is divided into modules, each module covers a single problem in bioinformatics that will be motivated based on current research. The module will then cover algorithms that solve the problem and variations on the problem. The problems covered are motif finding, edit distance in strings, sequence alignment, clustering, sequencing and assembly and finally computational methods for high throughput sequencing (HTS).
Face-to-face learningPrerequisitesSecond year- Fall
- STÆ312MApplied Linear Statistical ModelsMandatory (required) course6A mandatory (required) course for the programme6 ECTS, creditsCourse Description
The course focuses on simple and multiple linear regression as well as analysis of variance (ANOVA), analysis of covariance (ANCOVA) and binomial regression. The course is a natural continuation of a typical introductory course in statistics taught in various departments of the university.
We will discuss methods for estimating parameters in linear models, how to construct confidence intervals and test hypotheses for the parameters, which assumptions need to hold for applying the models and what to do when they are not met.
Students will work on projects using the statistical software R.
Face-to-face learningPrerequisitesLÝÐ301FBiostatistics II (Clinical Prediction Models )Mandatory (required) course6A mandatory (required) course for the programme6 ECTS, creditsCourse DescriptionThis course is a continuation of Biostatistics I and constitutes a practical guide to statistical analyses of student's own research projects. The course covers the following topics. Estimation of relative risk/odds ratios and adjusted estimation of relative risk/odds ratios, correlation and simple linear regression, multiple linear regression and logistic regression. The course is based on lectures and practical sessions using R for statistical analyses.
Face-to-face learningPrerequisitesCourse taught first half of the semesterLÝÐ108FOrientation seminar: public health, epidemiology and biostatisticsMandatory (required) course1A mandatory (required) course for the programme1 ECTS, creditsCourse DescriptionThis is a preparatory course for students in these interdisciplinary and research based studies. The course covers various practical issues, methods, study planning, refernce search, and scientific literacy e.g.. Students also aquire basic training in the statistical program R.
Face-to-face learningPrerequisitesAttendance required in classLÝÐ107FEpidemiology - a quantitative methodologyMandatory (required) course6A mandatory (required) course for the programme6 ECTS, creditsCourse DescriptionThe course is an introduction to epidemiological research methods and causal inference. An overview is provided on measure of disease occurrence, measures of outcome (relative risks), and study design (experiments, intervention studies, cohort studies and case-control studies). Emphasis is on systematic errors and on methods to avoid such errors in planning (study design) and in data analyses. Students get training in reviewing epidemiological studies.
Face-to-face learningPrerequisitesMAS102MR ProgrammingMandatory (required) course3A mandatory (required) course for the programme3 ECTS, creditsCourse DescriptionStudents will perform traditional statistical analysis on real data sets. Special focus will be on regression methods, including multiple regression analysis. Students will apply sophisticated methods of graphical representation and automatic reporting. Students will hand in a projects where they apply the above mentioned methods on real datasets in order to answer research questions
Face-to-face learningPrerequisites- Spring 2
LÝÐ079FBiostatistics III (Survival analysis)Mandatory (required) course6A mandatory (required) course for the programme6 ECTS, creditsCourse DescriptionThe course covers methods for analysis of cohort studies using methods for time to event or survival analysis. It is based on the course Biostat III – Survival analysis for epidemiologists in R at the Karolinska Institutet: See (https://biostat3.net/index.html): "Topics covered include methods for estimating patient survival (life table and Kaplan-Meier methods), comparing survival between patient subgroups (log-rank test), and modelling survival (primarily Poisson regression, Cox proportional hazards model and flexible parametric models). The course addresses the concept of 'time' as a potential confounder or effect modifier and approaches to defining 'time' (e.g., time since entry, attained age, calendar time). The course will emphasise the basic concepts of statistical modelling in epidemiology, such as controlling for confounding and assessing effect modification."
Face-to-face learningPrerequisitesNot taught this semesterMAS201MStatistical ConsultingMandatory (required) course6A mandatory (required) course for the programme6 ECTS, creditsCourse DescriptionParticipants in the course will obtain training in practical statistics as used when providing general statistical counselling. The participants will be introduced to actual statistical projects by assisting students in various departments within the university. The participants will report on the projects in class, discuss options for solving the projects and subsequently assist the students with analyses using R and interpretation of results.
Face-to-face learningPrerequisitesLÝÐ202FThe Scientific Process: Ethics, Communication and PracticalitiesMandatory (required) course6A mandatory (required) course for the programme6 ECTS, creditsCourse DescriptionThe course constitutes a practical guide to the preparation of a health-related research study. Modules include: reference search and handling, development of hypotheses, creation of a systematic critical review within chosen field of research, development and presentation of research proposals.
The course is for graduate students who have chosen a field/research question for their dissertation project.
Students in MPH Public Health Sciences and MS Epidemiology and Biostatistics must pass the course before starting work on their final thesis.
Face-to-face learningPrerequisitesCourse taught second half of the semester- Whole year courses
- LÝÐ098FResearch Training in Public Health SciencesElective course8Free elective course within the programme8 ECTS, creditsCourse Description
The aim of the course is for students to gain training in methods and insight into the implementation of a specific research project in public health. Students get to know the theoretical background of the research they are working on and are trained to participate in a defined part of it, but the topics depend on the needs of the research project that is assigned. An example would be e.g. participation in the preparation of a research project and various work related to data collection and data processing (cleaning and/or analysis). Students will also get to know the different aspects of the research project under the guidance of the scientific staff leading the research and in collaboration with the research team.
Students attend regular meetings with the researchers and supervisors of the course. The research training is planned specifically for each student with regard to the content and progress of the research, the supervisor and the student's background. Study space is limited by the research projects that are ongoing at the Center for Public Health Sciences at any given time, and students apply for registration for the course at the program office.
Face-to-face learningPrerequisites- Fall
LÝÐ060LThesis in Epidemiology and BiostatisticsMandatory (required) course30A mandatory (required) course for the programme30 ECTS, creditsCourse DescriptionThe MS program in Epidemiology and Biostatistics is a 120 credit post-graduate and cross-disciplinary program, including a 30 or 60 credit research thesis. Projects that have been/could be submitted for publication can be awarded 60 credits. During the first semester students develop their research questions and choose a thesis advisor. A complete research proposal is presented at the end of the second semester. Departmental registration is determined by the students' area of interest and host faculty of their main advisor.
The objective of the MS thesis is to provide training in constructing, organising, developing and conducting a research or developmental project in the public health sciences and an understanding of the technical limits, regulations, ethics and laws that must be observed in such work. Students should be able to define and present research questions and propose original hypotheses.
Self-studyPrerequisitesPart of the total project/thesis credits- Spring 2
LÝÐ060LThesis in Epidemiology and BiostatisticsMandatory (required) course30A mandatory (required) course for the programme30 ECTS, creditsCourse DescriptionThe MS program in Epidemiology and Biostatistics is a 120 credit post-graduate and cross-disciplinary program, including a 30 or 60 credit research thesis. Projects that have been/could be submitted for publication can be awarded 60 credits. During the first semester students develop their research questions and choose a thesis advisor. A complete research proposal is presented at the end of the second semester. Departmental registration is determined by the students' area of interest and host faculty of their main advisor.
The objective of the MS thesis is to provide training in constructing, organising, developing and conducting a research or developmental project in the public health sciences and an understanding of the technical limits, regulations, ethics and laws that must be observed in such work. Students should be able to define and present research questions and propose original hypotheses.
Self-studyPrerequisitesPart of the total project/thesis credits- Fall
- LÝÐ101FPublic Health: Science, Politics, PreventionRestricted elective course6Restricted elective course, conditions apply6 ECTS, creditsCourse Description
The course provides an overview of definitions, history, aims, legislation, methods and ethical considerations in public health and public health sciences. The course lays emphasis on global public health as well as on the Icelandic health care system, its administration and funding in comparison with health care systems in other nations. An overview is provided on Icelandic and international databases on health and disease and possibilities for their utilization in research and policy making for health promotion. In addition, current public health issues at each time are emphasized.
Face-to-face learningPrerequisitesAttendance required in classCourse taught first half of the semesterSTÆ529MBayesian Data AnalysisRestricted elective course8Restricted elective course, conditions apply8 ECTS, creditsCourse DescriptionGoal: To train students in applying methods of Bayesian statistics for analysis of data. Topics: Theory of Bayesian inference, prior distributions, data distributions and posterior distributions. Bayesian inference for parameters of univariate and multivariate distributions: binomial; normal; Poisson; exponential; multivariate normal; multinomial. Model checking and model comparison: Bayesian p-values; deviance information criterion (DIC). Bayesian computation: Markov chain Monte Carlo (MCMC) methods; the Gibbs sampler; the Metropolis-Hastings algorithm; convergence diagnostistics. Linear models: normal linear models; hierarchical linear models; generalized linear models. Emphasis on data analysis using software, e.g. Matlab and R.
Face-to-face learningThe course is taught if the specified conditions are metPrerequisitesLÝÐ0A1FMixed Linear ModelsRestricted elective course6Restricted elective course, conditions apply6 ECTS, creditsCourse DescriptionThe course is about the theory and application of random effects, or linear mixed models, and related models for correlated response variables. The course will cover methods for continuous and approximately normally distributed variables. A statistical model for such data has to describe both the expected value and the covariance between observations. The theory extends the theory of general linear models. Special software is needed for such an analysis and the necessary packages are provided in R, STATA, and SAS. The application will be based on R but other programs will be introduced for comparison.
The course will be taught between beginning of September and end of November, meeting once a week. The teaching will be in a flipped class manner. All the material (both notes and lectures) are online (see below) and it is expected that students will have viewed the material before class. In class the theory will be discussed and then students are expected to work on the application on their own computer.Face-to-face learningPrerequisitesLÝÐ097FTopics in Epidemiology (Epidemiology III)Restricted elective course6Restricted elective course, conditions apply6 ECTS, creditsCourse DescriptionThe aim of the course is to increase students' understanding of different areas within epidemiology, provide an introduction to area-specific methods, and to enhance students' ability to interpret results and assess the quality of scientific research in epidemiology.
The course will cover 4-6 specific areas or topics within epidemiology. Examples include perinatal, nutritional, pharmacological, and infectious disease epidemiology; featured topics may vary from year to year.
Face-to-face learningPrerequisitesSTÆ313MTheoretical StatisticsRestricted elective course10Restricted elective course, conditions apply10 ECTS, creditsCourse DescriptionLikelihood, Sufficient Statistic, Sufficiency Principle, Nuisance Parameter, Conditioning Principle, Invariance Principle, Likelihood Theory. Hypothesis Testing, Simple and Composite Hypothesis, The Neyman-Pearson Lemma, Power, UMP-Test, Invariant Tests. Permutation Tests, Rank Tests. Interval Estimation, Confidence Interval, Confidence, Confidence Region. Point Estimation, Bias, Mean Square Error. Assignments are returned using LaTeX and consitute 20% of the final grade.
Face-to-face learningOnline learningThe course is taught if the specified conditions are metPrerequisitesSÁL138FLatent variable models IRestricted elective course8Restricted elective course, conditions apply8 ECTS, creditsCourse DescriptionThe course covers models used to work with the underlying variables in psychological measurements will be introduced. In the first course (Models for underlying variables I) we will work with confirmatory factor models and structural equation models (also known as path models). We will cover the assumptions of the models, how to work with them, and interpretation of results. Methods to work with different types of data will be discussed. In the second course (Models for underlying variables II) we will start by introducing methods for categorical variables and then move to the closely related item response models. Primary focus will be on models for binary data but the most common models for categorical data will be introduced. In the second part of the course, we will move on to models for longitudinal data that use underlying variables: latent growth models, cross-lagged product models, and models for intensive longitudinal methods (also known as Daily-diary data, Ambulatory assessment, or Ecological-momentary data). Emphasis will be on practical training in analyzing data with models through projects, as well as the theoretical basis of models.
Face-to-face learningPrerequisitesNot taught this semesterLÍF127FBiometryRestricted elective course8Restricted elective course, conditions apply8 ECTS, creditsCourse DescriptionNumerical methods are an essential part of biology and are applied to design of experiments and observations, description of result and their analysis. Sudents learn these methods by working on biological data and to interpretate its results. Main method include the maximum likelihood estimation, linear models, regression and analysis of variance and generalized linear models. Multivariate analysis. Bootstrap and permutation analysis. The analysis will done using R. The students will obtain an extensive exercise in applyin R on various biological datasets. Analysis of own data or an extensive dataset, presented in a report and a lecture.
Assessment: Written examen 50%, assignments, report and lecture (50%).
Face-to-face learningPrerequisitesCourse taught in period ISÁL139FConstruction of self report scalesElective course8Free elective course within the programme8 ECTS, creditsCourse DescriptionThis course is designed to introduce students to the practice of psychological scale development and testing. Classical test theory is introduced with an emphasis on understanding statistical concepts related to scale construction. The main focus of the course is on practical training in scale development and the controversial issues related to developing a psychological scale from scratch.
Face-to-face learningPrerequisitesNot taught this semesterLÍF513MHuman GeneticsElective course6Free elective course within the programme6 ECTS, creditsCourse DescriptionLectures: Mendelian genetics, organization of the human genome, structure of chromosomes, chromosomal changes and syndromes, gene mapping via association and whole genome sequencing methods, genetic analysis, genetic screening, genetics of simple and complex traits, genes and environment, cancer genetics, gene therapy, human and primate evolution, ethical issues concerning human genetics, informed consent and private information. Students are expected to have prior knowledge of the principles genetics.
Practical: Analyses of genetic data, study of chromosomal labelling, analyses of genetic associations and transcriptomes.
Face-to-face learningPrerequisitesAttendance required in classIÐN113FTime Series AnalysisElective course7,5Free elective course within the programme7,5 ECTS, creditsCourse DescriptionARMAX and other similar time series models. Non-stationary time series. Correlation and spectral analysis. Parameter estimation, parametric and non-parametric approaches, Least Squares and Maximum Likelihood. Model validation methods. Models with time dependent parameters. Numerical methods for minimization. Outlier detection and interpolation. Introduction to nonlinear time series models. Discrete state space models. Discrete state space models. Extensive use of MATLAB, especially the System Identification Toolbox.
Distance learningSelf-studyPrerequisitesNot taught this semesterSTÆ310MTheory of linear modelsElective course6Free elective course within the programme6 ECTS, creditsCourse DescriptionSimple and multiple linear regression, analysis of variance and covariance, inference, variances and covariances of estimators, influence and diagnostic analyses using residual and influence measures, simultaneous inference. General linear models as projections with ANOVA as special case, simultaneous inference of estimable functions. R is used in assignments. Solutions to assignments are returned in LaTeX and PDF format.
In addition selected topics will be visited, e.g. generalized linear models (GLMs), nonlinear regression and/or random/mixed effects models and/or bootstrap methods etc.
Students will present solutions to individually assigned
projects/exercises, each of which is handed in earlier through a web-page.This course is taught in semesters of even-numbered years.
Face-to-face learningOnline learningThe course is taught if the specified conditions are metPrerequisitesSTÆ012FComputing and Calculus for Applied StatisticsElective course8Free elective course within the programme8 ECTS, creditsCourse DescriptionUnivariate calculus (basic algebra, functi ons, polynomials, logarithms and exponenti al functi ons, conti nuity and limits, diff erenti ati on, local extrema andintegrati on).
Linear algebra (vectors, matrices, linear projecti ons with matrices, matrix inverses and determinants).
Programming in R (arithmeti c, functi ons and organizing R code).
Multi variate calculus (Jacobian, Hessian and double integrals).
The approach will be to address each mathemati cs topic using a mix of (a) basic theory (in the form of concepts rather than proofs), (b) computerprogramming using R to visualize the theory, and (c) examples exclusively from stati stics. Formal lectures are not planned, but students will be able toseek assistance with their weekly assignments.
The goal of the course is to cover the calculus, linear algebra and computer programming concepts most commonly needed in stati sti cs. A student who has completed this course should have the mathematical basis for statistics courses currently taught at the MSc level by the mathematics department(and thus also for all stati sti cs courses taught by other departments).Students will author examples and multiple-choice questi ons, and their submissions will be graded by their peers.
The material is openly available at https://open-educati on-hub.github.io/ccas/.
Face-to-face learningPrerequisites- Spring 2
LÝÐ085FEpidemiologic Methods (Epidemiology II)Restricted elective course6Restricted elective course, conditions apply6 ECTS, creditsCourse DescriptionThe aim of the course is to increase students‘ understanding of advanced methods in epidemiology and to enhance students‘ ability to interpret results and assess the quality of scientific research in epidemiology.
The course will cover positive and negative confounding, matching, propensity score, effect modification and interaction, instrumental variables, causal diagrams, and missing data. Scientific articles in epidemiology will be studied and discussed.
Face-to-face learningPrerequisitesSTÆ004FRandom Effects ModelsRestricted elective course8Restricted elective course, conditions apply8 ECTS, creditsCourse DescriptionThe focus of this course is on Bayesian latent Gaussian models (BLGMs) which are a class of Bayesian hierarchical models and applications of these models. The main topics are three types of BLGMs: (i) Bayesian Gaussian—Gaussian models, (ii) BLGMs with a univariate link function, and (iii) BLGMs with a multivariate link function, as well as prior densities for BLGMs and posterior computation for BLGMs. In the first part of the course, the basics of these models is covered and homework assignments will be given on these topics. In the second part of the course, the focus is on a project, in which data are analyzed using BLGMs. Each student can contribute data that she or he wishes to analyze. The material in the course is based on a theoretical background. However, the focus on data analysis is strong, and computation and programming play a large role in the course. Thus, the course will be useful to students in their future projects involving data analysis.
Linear regression models, the multiple normal distribution, hierarchical models, fixed and random effect models, restricted maximum likelihood estimation, best linear unbiased estimators, Bayesian inference, statistical decision theory, Markov chains, Monte Carlo integration, importance sampling, Markov chain Monte Carlo, Gibbs sampling, the Metropolis-Hastings algorithm.
Face-to-face learningPrerequisitesNot taught this semesterSTÆ415MStochastic ProcessesElective course10Free elective course within the programme10 ECTS, creditsCourse DescriptionIntroduction to stochastic processes with main emphasis on Markov chains.
Subject matter: Hitting time, classification of states, irreducibility, period, recurrence (positive and null), transience, regeneration, coupling, stationarity, time-reversibility, coupling from the past, branching processes, queues, martingales, Brownian motion.Face-to-face learningThe course is taught if the specified conditions are metPrerequisitesLÝÐ0A0FTrauma and its impact on healthElective course6Free elective course within the programme6 ECTS, creditsCourse DescriptionThis course describes trauma in childhood and adulthood, including violence, accidents, disasters and life-threatening illness and their association with mental and physical health. Emphasis will be placed on introducing the scientific foundation of the trauma field and understanding scientific articles in this area. The main topics of the course include:
- Prevalence of traumatic events and acute stress reactions.
- Mental health problems following trauma, such as posttraumatic stress disorder, anxiety, depression, sleep disturbances, substance abuse and prolonged grief.
- The disease burden of trauma, due to e.g., cardiovascular diseases, cancers, autoimmune diseases, and suicide.
- The influence of environmental and genetic factors in the development of psychological and physical diseases following trauma.
- Factors that promote recovery post-trauma and reduce the risk of long-term health problems.
- Evidence-based treatment options for PTSD.
The course is intended for students who want to increase their scientific knowledge of the relationship between trauma and health. It is only intended for postgraduate students. The course consists of lectures by the main supervisor and selected guest speakers who are experts in the field of trauma. Emphasis will be placed on discussions and active participation of students.
Distance learningPrerequisitesAttendance required in classCourse taught second half of the semesterMAS202MApplied data analysisElective course6Free elective course within the programme6 ECTS, creditsCourse DescriptionThe course focuses on statistical analysis using the R environment. It is assumed that students have basic knowledge of statistics and the statistical software R. Students will learn to apply a broad range of statistical methods in R (such as classification methods, resampling methods, linear model selection and tree-based methods). The course on 12 weeks and will be on "flipped" form. This means that no lectures will be given but students will read some material and watch videos before attending classes. Students will then work on assignments during the classes.
Face-to-face learningPrerequisitesNÆR506MApplied multivariable regression and data analysisElective course6Free elective course within the programme6 ECTS, creditsCourse DescriptionThe aim of this course is to enable student to conduct their own data analyses. This includes familiarizing them with practical aspects of data cleaning/processing and statistical methods used within nutritional epidemiology.
Short lectures will be given covering selected subjects followed by practical assignments. Assignments will contribute 100% to the final grade.
Some experience with SPSS, SAS or related softwere in addition to having taken basic course in statistics is desierable, but not required.
Face-to-face learningPrerequisitesFMÞ501MSocial science statistics: Regression analysisElective course10Free elective course within the programme10 ECTS, creditsCourse DescriptionThis is a comprehensive course in multiple-regression analysis. The goal of the course is that students develop enough conceptual understanding and practical knowledge to use this method on their own. The lectures cover various regression analysis techniques commonly used in quantitative social research, including control variables, the use of nominal variables, linear and nonlinear models, techniques that test for mediation and statistical interaction effects, and so on. We discuss the assumptions of regression analysis and learn techniques to detect and deal with violations of assumptions. In addition, logistic regression will be introduced, which is a method for a dichotomous dependent variable. We also review many of the basic concepts involved in statistical inference and significance testing. Students get plenty of hands-on experience with data analysis. The instructor hands out survey data that students use to practice the techniques covered in class. The statistical package SPSS will be used.
Face-to-face learningPrerequisitesLÍF659MGenomics and bioinformaticsElective course6Free elective course within the programme6 ECTS, creditsCourse DescriptionGenomics and bioinformatics are intertwinned in many ways. Technological advances enabled the sequencing of for instance genomes, transcriptomes and proteomes. Complete genome sequences of thousands of organisms enables study of this flood of information for gaining knowledge and deeper understanding of biological phenomena. Comparative studies, in one way or another, building on Darwininan thought provide the theoretical underpinnings for analyzing this information and it applications. Characters and features conserved among organisms are based in conserved parts of genomes and conversely, new and unique phenotypes are affected by variable parts of genomes. This applies equally to animals, plants and microbes, and cells, enzymatic and developmental systems.
The course centers on the theoretical and practical aspects of comparative analysis, about analyses of genomes, metagenomes and transcriptomes to study biological, medical and applied questions. The lectures cover structure and sequencing of genomes, transcriptomes and proteomes, molecular evolution, different types of bioinformatic data, shell scripts, intro to R and Python scripting and applications. The practicals include, retrieval of data from databases, blast and alignment, assembly and annotation, comparison of genomes, population data analyses. Students will work with databases, such as Flybase, Genebank and ENSEMBL. Data will be retrived with Biomart and Bioconductor, and data quality discussed. Algorithms for search tools and alignments, read counts and comparisons of groups and treatments. Also elements of python scripting, open linux software, installation of linux programs, analyses of data from RNA-seq, RADseq and genome sequencing.
Students are required to turn in a few small and one big group project and present the large project with a lecture. In discussion session primary literature will be presented.Face-to-face learningDistance learningThe course is taught if the specified conditions are metPrerequisitesCourse DescriptionLectures: The molecular basis of life (chemical bonds, biological molecules, structure of DNA, RNA and proteins). Genomes and the flow of biological information. Chromosome structure and function, chromatin and nucleosomes. The cell cycle, DNA replication. Chromosome segregaition, Transcription. Regulation of transcription. RNA processing. Translation. Regulation of translation. Regulatory RNAs. Protein modification and targeting. DNA damage, checkpoints and DNA repair mechanisms. Repair of DNA double-strand breaks and homologous recombination. Mobile DNA elements. Tools and techniques in molecular Biology icluding Model organisms.
Seminar: Students present and discuss selected research papers and hand in a short essay.
Laboratory work: Work on molecular genetics project relevant to current research. Basic methods such as gene cloning, gene transfer and expression, PCR, sequencing, DNA isolation and restriction analysis, electrophoresis of DNA and proteins will be used.
Exam: Laboratory 10%, seminar 15%, written final exam 75%.
Face-to-face learningPrerequisitesSTÆ418MIntroduction to Measure-Theoretic ProbabilityElective course10Free elective course within the programme10 ECTS, creditsCourse DescriptionProbability based on measure-theory.
Subject matter: Probability, extension theorems, independence, expectation. The Borel-Cantelli theorem and the Kolmogorov 0-1 law. Inequalities and the weak and strong laws of large numbers. Convergence pointwise, in probability, with probability one, in distribtution, and in total variation. Coupling methods. The central limit theorem. Conditional probability and expectation.
Face-to-face learningThe course is taught if the specified conditions are metPrerequisitesLÍF659MGenomics and bioinformaticsElective course6Free elective course within the programme6 ECTS, creditsCourse DescriptionGenomics and bioinformatics are intertwinned in many ways. Technological advances enabled the sequencing of for instance genomes, transcriptomes and proteomes. Complete genome sequences of thousands of organisms enables study of this flood of information for gaining knowledge and deeper understanding of biological phenomena. Comparative studies, in one way or another, building on Darwininan thought provide the theoretical underpinnings for analyzing this information and it applications. Characters and features conserved among organisms are based in conserved parts of genomes and conversely, new and unique phenotypes are affected by variable parts of genomes. This applies equally to animals, plants and microbes, and cells, enzymatic and developmental systems.
The course centers on the theoretical and practical aspects of comparative analysis, about analyses of genomes, metagenomes and transcriptomes to study biological, medical and applied questions. The lectures cover structure and sequencing of genomes, transcriptomes and proteomes, molecular evolution, different types of bioinformatic data, shell scripts, intro to R and Python scripting and applications. The practicals include, retrieval of data from databases, blast and alignment, assembly and annotation, comparison of genomes, population data analyses. Students will work with databases, such as Flybase, Genebank and ENSEMBL. Data will be retrived with Biomart and Bioconductor, and data quality discussed. Algorithms for search tools and alignments, read counts and comparisons of groups and treatments. Also elements of python scripting, open linux software, installation of linux programs, analyses of data from RNA-seq, RADseq and genome sequencing.
Students are required to turn in a few small and one big group project and present the large project with a lecture. In discussion session primary literature will be presented.Face-to-face learningDistance learningThe course is taught if the specified conditions are metPrerequisitesTÖL604MAlgorithms in BioinformaticsElective course6Free elective course within the programme6 ECTS, creditsCourse DescriptionThis course will cover the algorithmic aspects of bioinformatic. We will start with an introduction to genomics and algorithms for students new to the field. The course is divided into modules, each module covers a single problem in bioinformatics that will be motivated based on current research. The module will then cover algorithms that solve the problem and variations on the problem. The problems covered are motif finding, edit distance in strings, sequence alignment, clustering, sequencing and assembly and finally computational methods for high throughput sequencing (HTS).
Face-to-face learningPrerequisitesYear unspecified- Fall
- STÆ312MApplied Linear Statistical ModelsMandatory (required) course6A mandatory (required) course for the programme6 ECTS, creditsCourse Description
The course focuses on simple and multiple linear regression as well as analysis of variance (ANOVA), analysis of covariance (ANCOVA) and binomial regression. The course is a natural continuation of a typical introductory course in statistics taught in various departments of the university.
We will discuss methods for estimating parameters in linear models, how to construct confidence intervals and test hypotheses for the parameters, which assumptions need to hold for applying the models and what to do when they are not met.
Students will work on projects using the statistical software R.
Face-to-face learningPrerequisitesLÝÐ301FBiostatistics II (Clinical Prediction Models )Mandatory (required) course6A mandatory (required) course for the programme6 ECTS, creditsCourse DescriptionThis course is a continuation of Biostatistics I and constitutes a practical guide to statistical analyses of student's own research projects. The course covers the following topics. Estimation of relative risk/odds ratios and adjusted estimation of relative risk/odds ratios, correlation and simple linear regression, multiple linear regression and logistic regression. The course is based on lectures and practical sessions using R for statistical analyses.
Face-to-face learningPrerequisitesCourse taught first half of the semesterLÝÐ108FOrientation seminar: public health, epidemiology and biostatisticsMandatory (required) course1A mandatory (required) course for the programme1 ECTS, creditsCourse DescriptionThis is a preparatory course for students in these interdisciplinary and research based studies. The course covers various practical issues, methods, study planning, refernce search, and scientific literacy e.g.. Students also aquire basic training in the statistical program R.
Face-to-face learningPrerequisitesAttendance required in classLÝÐ107FEpidemiology - a quantitative methodologyMandatory (required) course6A mandatory (required) course for the programme6 ECTS, creditsCourse DescriptionThe course is an introduction to epidemiological research methods and causal inference. An overview is provided on measure of disease occurrence, measures of outcome (relative risks), and study design (experiments, intervention studies, cohort studies and case-control studies). Emphasis is on systematic errors and on methods to avoid such errors in planning (study design) and in data analyses. Students get training in reviewing epidemiological studies.
Face-to-face learningPrerequisitesMAS102MR ProgrammingMandatory (required) course3A mandatory (required) course for the programme3 ECTS, creditsCourse DescriptionStudents will perform traditional statistical analysis on real data sets. Special focus will be on regression methods, including multiple regression analysis. Students will apply sophisticated methods of graphical representation and automatic reporting. Students will hand in a projects where they apply the above mentioned methods on real datasets in order to answer research questions
Face-to-face learningPrerequisites- Spring 2
LÝÐ079FBiostatistics III (Survival analysis)Mandatory (required) course6A mandatory (required) course for the programme6 ECTS, creditsCourse DescriptionThe course covers methods for analysis of cohort studies using methods for time to event or survival analysis. It is based on the course Biostat III – Survival analysis for epidemiologists in R at the Karolinska Institutet: See (https://biostat3.net/index.html): "Topics covered include methods for estimating patient survival (life table and Kaplan-Meier methods), comparing survival between patient subgroups (log-rank test), and modelling survival (primarily Poisson regression, Cox proportional hazards model and flexible parametric models). The course addresses the concept of 'time' as a potential confounder or effect modifier and approaches to defining 'time' (e.g., time since entry, attained age, calendar time). The course will emphasise the basic concepts of statistical modelling in epidemiology, such as controlling for confounding and assessing effect modification."
Face-to-face learningPrerequisitesNot taught this semesterMAS201MStatistical ConsultingMandatory (required) course6A mandatory (required) course for the programme6 ECTS, creditsCourse DescriptionParticipants in the course will obtain training in practical statistics as used when providing general statistical counselling. The participants will be introduced to actual statistical projects by assisting students in various departments within the university. The participants will report on the projects in class, discuss options for solving the projects and subsequently assist the students with analyses using R and interpretation of results.
Face-to-face learningPrerequisitesLÝÐ202FThe Scientific Process: Ethics, Communication and PracticalitiesMandatory (required) course6A mandatory (required) course for the programme6 ECTS, creditsCourse DescriptionThe course constitutes a practical guide to the preparation of a health-related research study. Modules include: reference search and handling, development of hypotheses, creation of a systematic critical review within chosen field of research, development and presentation of research proposals.
The course is for graduate students who have chosen a field/research question for their dissertation project.
Students in MPH Public Health Sciences and MS Epidemiology and Biostatistics must pass the course before starting work on their final thesis.
Face-to-face learningPrerequisitesCourse taught second half of the semester- Whole year courses
- LÝÐ098FResearch Training in Public Health SciencesElective course8Free elective course within the programme8 ECTS, creditsCourse Description
The aim of the course is for students to gain training in methods and insight into the implementation of a specific research project in public health. Students get to know the theoretical background of the research they are working on and are trained to participate in a defined part of it, but the topics depend on the needs of the research project that is assigned. An example would be e.g. participation in the preparation of a research project and various work related to data collection and data processing (cleaning and/or analysis). Students will also get to know the different aspects of the research project under the guidance of the scientific staff leading the research and in collaboration with the research team.
Students attend regular meetings with the researchers and supervisors of the course. The research training is planned specifically for each student with regard to the content and progress of the research, the supervisor and the student's background. Study space is limited by the research projects that are ongoing at the Center for Public Health Sciences at any given time, and students apply for registration for the course at the program office.
Face-to-face learningPrerequisites- Fall
LÝÐ060LThesis in Epidemiology and BiostatisticsMandatory (required) course30A mandatory (required) course for the programme30 ECTS, creditsCourse DescriptionThe MS program in Epidemiology and Biostatistics is a 120 credit post-graduate and cross-disciplinary program, including a 30 or 60 credit research thesis. Projects that have been/could be submitted for publication can be awarded 60 credits. During the first semester students develop their research questions and choose a thesis advisor. A complete research proposal is presented at the end of the second semester. Departmental registration is determined by the students' area of interest and host faculty of their main advisor.
The objective of the MS thesis is to provide training in constructing, organising, developing and conducting a research or developmental project in the public health sciences and an understanding of the technical limits, regulations, ethics and laws that must be observed in such work. Students should be able to define and present research questions and propose original hypotheses.
Self-studyPrerequisitesPart of the total project/thesis credits- Spring 2
LÝÐ060LThesis in Epidemiology and BiostatisticsMandatory (required) course30A mandatory (required) course for the programme30 ECTS, creditsCourse DescriptionThe MS program in Epidemiology and Biostatistics is a 120 credit post-graduate and cross-disciplinary program, including a 30 or 60 credit research thesis. Projects that have been/could be submitted for publication can be awarded 60 credits. During the first semester students develop their research questions and choose a thesis advisor. A complete research proposal is presented at the end of the second semester. Departmental registration is determined by the students' area of interest and host faculty of their main advisor.
The objective of the MS thesis is to provide training in constructing, organising, developing and conducting a research or developmental project in the public health sciences and an understanding of the technical limits, regulations, ethics and laws that must be observed in such work. Students should be able to define and present research questions and propose original hypotheses.
Self-studyPrerequisitesPart of the total project/thesis credits- Fall
- LÝÐ101FPublic Health: Science, Politics, PreventionRestricted elective course6Restricted elective course, conditions apply6 ECTS, creditsCourse Description
The course provides an overview of definitions, history, aims, legislation, methods and ethical considerations in public health and public health sciences. The course lays emphasis on global public health as well as on the Icelandic health care system, its administration and funding in comparison with health care systems in other nations. An overview is provided on Icelandic and international databases on health and disease and possibilities for their utilization in research and policy making for health promotion. In addition, current public health issues at each time are emphasized.
Face-to-face learningPrerequisitesAttendance required in classCourse taught first half of the semesterSTÆ529MBayesian Data AnalysisRestricted elective course8Restricted elective course, conditions apply8 ECTS, creditsCourse DescriptionGoal: To train students in applying methods of Bayesian statistics for analysis of data. Topics: Theory of Bayesian inference, prior distributions, data distributions and posterior distributions. Bayesian inference for parameters of univariate and multivariate distributions: binomial; normal; Poisson; exponential; multivariate normal; multinomial. Model checking and model comparison: Bayesian p-values; deviance information criterion (DIC). Bayesian computation: Markov chain Monte Carlo (MCMC) methods; the Gibbs sampler; the Metropolis-Hastings algorithm; convergence diagnostistics. Linear models: normal linear models; hierarchical linear models; generalized linear models. Emphasis on data analysis using software, e.g. Matlab and R.
Face-to-face learningThe course is taught if the specified conditions are metPrerequisitesLÝÐ0A1FMixed Linear ModelsRestricted elective course6Restricted elective course, conditions apply6 ECTS, creditsCourse DescriptionThe course is about the theory and application of random effects, or linear mixed models, and related models for correlated response variables. The course will cover methods for continuous and approximately normally distributed variables. A statistical model for such data has to describe both the expected value and the covariance between observations. The theory extends the theory of general linear models. Special software is needed for such an analysis and the necessary packages are provided in R, STATA, and SAS. The application will be based on R but other programs will be introduced for comparison.
The course will be taught between beginning of September and end of November, meeting once a week. The teaching will be in a flipped class manner. All the material (both notes and lectures) are online (see below) and it is expected that students will have viewed the material before class. In class the theory will be discussed and then students are expected to work on the application on their own computer.Face-to-face learningPrerequisitesLÝÐ097FTopics in Epidemiology (Epidemiology III)Restricted elective course6Restricted elective course, conditions apply6 ECTS, creditsCourse DescriptionThe aim of the course is to increase students' understanding of different areas within epidemiology, provide an introduction to area-specific methods, and to enhance students' ability to interpret results and assess the quality of scientific research in epidemiology.
The course will cover 4-6 specific areas or topics within epidemiology. Examples include perinatal, nutritional, pharmacological, and infectious disease epidemiology; featured topics may vary from year to year.
Face-to-face learningPrerequisitesSTÆ313MTheoretical StatisticsRestricted elective course10Restricted elective course, conditions apply10 ECTS, creditsCourse DescriptionLikelihood, Sufficient Statistic, Sufficiency Principle, Nuisance Parameter, Conditioning Principle, Invariance Principle, Likelihood Theory. Hypothesis Testing, Simple and Composite Hypothesis, The Neyman-Pearson Lemma, Power, UMP-Test, Invariant Tests. Permutation Tests, Rank Tests. Interval Estimation, Confidence Interval, Confidence, Confidence Region. Point Estimation, Bias, Mean Square Error. Assignments are returned using LaTeX and consitute 20% of the final grade.
Face-to-face learningOnline learningThe course is taught if the specified conditions are metPrerequisitesSÁL138FLatent variable models IRestricted elective course8Restricted elective course, conditions apply8 ECTS, creditsCourse DescriptionThe course covers models used to work with the underlying variables in psychological measurements will be introduced. In the first course (Models for underlying variables I) we will work with confirmatory factor models and structural equation models (also known as path models). We will cover the assumptions of the models, how to work with them, and interpretation of results. Methods to work with different types of data will be discussed. In the second course (Models for underlying variables II) we will start by introducing methods for categorical variables and then move to the closely related item response models. Primary focus will be on models for binary data but the most common models for categorical data will be introduced. In the second part of the course, we will move on to models for longitudinal data that use underlying variables: latent growth models, cross-lagged product models, and models for intensive longitudinal methods (also known as Daily-diary data, Ambulatory assessment, or Ecological-momentary data). Emphasis will be on practical training in analyzing data with models through projects, as well as the theoretical basis of models.
Face-to-face learningPrerequisitesNot taught this semesterLÍF127FBiometryRestricted elective course8Restricted elective course, conditions apply8 ECTS, creditsCourse DescriptionNumerical methods are an essential part of biology and are applied to design of experiments and observations, description of result and their analysis. Sudents learn these methods by working on biological data and to interpretate its results. Main method include the maximum likelihood estimation, linear models, regression and analysis of variance and generalized linear models. Multivariate analysis. Bootstrap and permutation analysis. The analysis will done using R. The students will obtain an extensive exercise in applyin R on various biological datasets. Analysis of own data or an extensive dataset, presented in a report and a lecture.
Assessment: Written examen 50%, assignments, report and lecture (50%).
Face-to-face learningPrerequisitesCourse taught in period ISÁL139FConstruction of self report scalesElective course8Free elective course within the programme8 ECTS, creditsCourse DescriptionThis course is designed to introduce students to the practice of psychological scale development and testing. Classical test theory is introduced with an emphasis on understanding statistical concepts related to scale construction. The main focus of the course is on practical training in scale development and the controversial issues related to developing a psychological scale from scratch.
Face-to-face learningPrerequisitesNot taught this semesterLÍF513MHuman GeneticsElective course6Free elective course within the programme6 ECTS, creditsCourse DescriptionLectures: Mendelian genetics, organization of the human genome, structure of chromosomes, chromosomal changes and syndromes, gene mapping via association and whole genome sequencing methods, genetic analysis, genetic screening, genetics of simple and complex traits, genes and environment, cancer genetics, gene therapy, human and primate evolution, ethical issues concerning human genetics, informed consent and private information. Students are expected to have prior knowledge of the principles genetics.
Practical: Analyses of genetic data, study of chromosomal labelling, analyses of genetic associations and transcriptomes.
Face-to-face learningPrerequisitesAttendance required in classIÐN113FTime Series AnalysisElective course7,5Free elective course within the programme7,5 ECTS, creditsCourse DescriptionARMAX and other similar time series models. Non-stationary time series. Correlation and spectral analysis. Parameter estimation, parametric and non-parametric approaches, Least Squares and Maximum Likelihood. Model validation methods. Models with time dependent parameters. Numerical methods for minimization. Outlier detection and interpolation. Introduction to nonlinear time series models. Discrete state space models. Discrete state space models. Extensive use of MATLAB, especially the System Identification Toolbox.
Distance learningSelf-studyPrerequisitesNot taught this semesterSTÆ310MTheory of linear modelsElective course6Free elective course within the programme6 ECTS, creditsCourse DescriptionSimple and multiple linear regression, analysis of variance and covariance, inference, variances and covariances of estimators, influence and diagnostic analyses using residual and influence measures, simultaneous inference. General linear models as projections with ANOVA as special case, simultaneous inference of estimable functions. R is used in assignments. Solutions to assignments are returned in LaTeX and PDF format.
In addition selected topics will be visited, e.g. generalized linear models (GLMs), nonlinear regression and/or random/mixed effects models and/or bootstrap methods etc.
Students will present solutions to individually assigned
projects/exercises, each of which is handed in earlier through a web-page.This course is taught in semesters of even-numbered years.
Face-to-face learningOnline learningThe course is taught if the specified conditions are metPrerequisitesSTÆ012FComputing and Calculus for Applied StatisticsElective course8Free elective course within the programme8 ECTS, creditsCourse DescriptionUnivariate calculus (basic algebra, functi ons, polynomials, logarithms and exponenti al functi ons, conti nuity and limits, diff erenti ati on, local extrema andintegrati on).
Linear algebra (vectors, matrices, linear projecti ons with matrices, matrix inverses and determinants).
Programming in R (arithmeti c, functi ons and organizing R code).
Multi variate calculus (Jacobian, Hessian and double integrals).
The approach will be to address each mathemati cs topic using a mix of (a) basic theory (in the form of concepts rather than proofs), (b) computerprogramming using R to visualize the theory, and (c) examples exclusively from stati stics. Formal lectures are not planned, but students will be able toseek assistance with their weekly assignments.
The goal of the course is to cover the calculus, linear algebra and computer programming concepts most commonly needed in stati sti cs. A student who has completed this course should have the mathematical basis for statistics courses currently taught at the MSc level by the mathematics department(and thus also for all stati sti cs courses taught by other departments).Students will author examples and multiple-choice questi ons, and their submissions will be graded by their peers.
The material is openly available at https://open-educati on-hub.github.io/ccas/.
Face-to-face learningPrerequisites- Spring 2
LÝÐ085FEpidemiologic Methods (Epidemiology II)Restricted elective course6Restricted elective course, conditions apply6 ECTS, creditsCourse DescriptionThe aim of the course is to increase students‘ understanding of advanced methods in epidemiology and to enhance students‘ ability to interpret results and assess the quality of scientific research in epidemiology.
The course will cover positive and negative confounding, matching, propensity score, effect modification and interaction, instrumental variables, causal diagrams, and missing data. Scientific articles in epidemiology will be studied and discussed.
Face-to-face learningPrerequisitesSTÆ004FRandom Effects ModelsRestricted elective course8Restricted elective course, conditions apply8 ECTS, creditsCourse DescriptionThe focus of this course is on Bayesian latent Gaussian models (BLGMs) which are a class of Bayesian hierarchical models and applications of these models. The main topics are three types of BLGMs: (i) Bayesian Gaussian—Gaussian models, (ii) BLGMs with a univariate link function, and (iii) BLGMs with a multivariate link function, as well as prior densities for BLGMs and posterior computation for BLGMs. In the first part of the course, the basics of these models is covered and homework assignments will be given on these topics. In the second part of the course, the focus is on a project, in which data are analyzed using BLGMs. Each student can contribute data that she or he wishes to analyze. The material in the course is based on a theoretical background. However, the focus on data analysis is strong, and computation and programming play a large role in the course. Thus, the course will be useful to students in their future projects involving data analysis.
Linear regression models, the multiple normal distribution, hierarchical models, fixed and random effect models, restricted maximum likelihood estimation, best linear unbiased estimators, Bayesian inference, statistical decision theory, Markov chains, Monte Carlo integration, importance sampling, Markov chain Monte Carlo, Gibbs sampling, the Metropolis-Hastings algorithm.
Face-to-face learningPrerequisitesNot taught this semesterSTÆ415MStochastic ProcessesElective course10Free elective course within the programme10 ECTS, creditsCourse DescriptionIntroduction to stochastic processes with main emphasis on Markov chains.
Subject matter: Hitting time, classification of states, irreducibility, period, recurrence (positive and null), transience, regeneration, coupling, stationarity, time-reversibility, coupling from the past, branching processes, queues, martingales, Brownian motion.Face-to-face learningThe course is taught if the specified conditions are metPrerequisitesLÝÐ0A0FTrauma and its impact on healthElective course6Free elective course within the programme6 ECTS, creditsCourse DescriptionThis course describes trauma in childhood and adulthood, including violence, accidents, disasters and life-threatening illness and their association with mental and physical health. Emphasis will be placed on introducing the scientific foundation of the trauma field and understanding scientific articles in this area. The main topics of the course include:
- Prevalence of traumatic events and acute stress reactions.
- Mental health problems following trauma, such as posttraumatic stress disorder, anxiety, depression, sleep disturbances, substance abuse and prolonged grief.
- The disease burden of trauma, due to e.g., cardiovascular diseases, cancers, autoimmune diseases, and suicide.
- The influence of environmental and genetic factors in the development of psychological and physical diseases following trauma.
- Factors that promote recovery post-trauma and reduce the risk of long-term health problems.
- Evidence-based treatment options for PTSD.
The course is intended for students who want to increase their scientific knowledge of the relationship between trauma and health. It is only intended for postgraduate students. The course consists of lectures by the main supervisor and selected guest speakers who are experts in the field of trauma. Emphasis will be placed on discussions and active participation of students.
Distance learningPrerequisitesAttendance required in classCourse taught second half of the semesterMAS202MApplied data analysisElective course6Free elective course within the programme6 ECTS, creditsCourse DescriptionThe course focuses on statistical analysis using the R environment. It is assumed that students have basic knowledge of statistics and the statistical software R. Students will learn to apply a broad range of statistical methods in R (such as classification methods, resampling methods, linear model selection and tree-based methods). The course on 12 weeks and will be on "flipped" form. This means that no lectures will be given but students will read some material and watch videos before attending classes. Students will then work on assignments during the classes.
Face-to-face learningPrerequisitesNÆR506MApplied multivariable regression and data analysisElective course6Free elective course within the programme6 ECTS, creditsCourse DescriptionThe aim of this course is to enable student to conduct their own data analyses. This includes familiarizing them with practical aspects of data cleaning/processing and statistical methods used within nutritional epidemiology.
Short lectures will be given covering selected subjects followed by practical assignments. Assignments will contribute 100% to the final grade.
Some experience with SPSS, SAS or related softwere in addition to having taken basic course in statistics is desierable, but not required.
Face-to-face learningPrerequisitesFMÞ501MSocial science statistics: Regression analysisElective course10Free elective course within the programme10 ECTS, creditsCourse DescriptionThis is a comprehensive course in multiple-regression analysis. The goal of the course is that students develop enough conceptual understanding and practical knowledge to use this method on their own. The lectures cover various regression analysis techniques commonly used in quantitative social research, including control variables, the use of nominal variables, linear and nonlinear models, techniques that test for mediation and statistical interaction effects, and so on. We discuss the assumptions of regression analysis and learn techniques to detect and deal with violations of assumptions. In addition, logistic regression will be introduced, which is a method for a dichotomous dependent variable. We also review many of the basic concepts involved in statistical inference and significance testing. Students get plenty of hands-on experience with data analysis. The instructor hands out survey data that students use to practice the techniques covered in class. The statistical package SPSS will be used.
Face-to-face learningPrerequisitesLÍF659MGenomics and bioinformaticsElective course6Free elective course within the programme6 ECTS, creditsCourse DescriptionGenomics and bioinformatics are intertwinned in many ways. Technological advances enabled the sequencing of for instance genomes, transcriptomes and proteomes. Complete genome sequences of thousands of organisms enables study of this flood of information for gaining knowledge and deeper understanding of biological phenomena. Comparative studies, in one way or another, building on Darwininan thought provide the theoretical underpinnings for analyzing this information and it applications. Characters and features conserved among organisms are based in conserved parts of genomes and conversely, new and unique phenotypes are affected by variable parts of genomes. This applies equally to animals, plants and microbes, and cells, enzymatic and developmental systems.
The course centers on the theoretical and practical aspects of comparative analysis, about analyses of genomes, metagenomes and transcriptomes to study biological, medical and applied questions. The lectures cover structure and sequencing of genomes, transcriptomes and proteomes, molecular evolution, different types of bioinformatic data, shell scripts, intro to R and Python scripting and applications. The practicals include, retrieval of data from databases, blast and alignment, assembly and annotation, comparison of genomes, population data analyses. Students will work with databases, such as Flybase, Genebank and ENSEMBL. Data will be retrived with Biomart and Bioconductor, and data quality discussed. Algorithms for search tools and alignments, read counts and comparisons of groups and treatments. Also elements of python scripting, open linux software, installation of linux programs, analyses of data from RNA-seq, RADseq and genome sequencing.
Students are required to turn in a few small and one big group project and present the large project with a lecture. In discussion session primary literature will be presented.Face-to-face learningDistance learningThe course is taught if the specified conditions are metPrerequisitesCourse DescriptionLectures: The molecular basis of life (chemical bonds, biological molecules, structure of DNA, RNA and proteins). Genomes and the flow of biological information. Chromosome structure and function, chromatin and nucleosomes. The cell cycle, DNA replication. Chromosome segregaition, Transcription. Regulation of transcription. RNA processing. Translation. Regulation of translation. Regulatory RNAs. Protein modification and targeting. DNA damage, checkpoints and DNA repair mechanisms. Repair of DNA double-strand breaks and homologous recombination. Mobile DNA elements. Tools and techniques in molecular Biology icluding Model organisms.
Seminar: Students present and discuss selected research papers and hand in a short essay.
Laboratory work: Work on molecular genetics project relevant to current research. Basic methods such as gene cloning, gene transfer and expression, PCR, sequencing, DNA isolation and restriction analysis, electrophoresis of DNA and proteins will be used.
Exam: Laboratory 10%, seminar 15%, written final exam 75%.
Face-to-face learningPrerequisitesSTÆ418MIntroduction to Measure-Theoretic ProbabilityElective course10Free elective course within the programme10 ECTS, creditsCourse DescriptionProbability based on measure-theory.
Subject matter: Probability, extension theorems, independence, expectation. The Borel-Cantelli theorem and the Kolmogorov 0-1 law. Inequalities and the weak and strong laws of large numbers. Convergence pointwise, in probability, with probability one, in distribtution, and in total variation. Coupling methods. The central limit theorem. Conditional probability and expectation.
Face-to-face learningThe course is taught if the specified conditions are metPrerequisitesLÍF659MGenomics and bioinformaticsElective course6Free elective course within the programme6 ECTS, creditsCourse DescriptionGenomics and bioinformatics are intertwinned in many ways. Technological advances enabled the sequencing of for instance genomes, transcriptomes and proteomes. Complete genome sequences of thousands of organisms enables study of this flood of information for gaining knowledge and deeper understanding of biological phenomena. Comparative studies, in one way or another, building on Darwininan thought provide the theoretical underpinnings for analyzing this information and it applications. Characters and features conserved among organisms are based in conserved parts of genomes and conversely, new and unique phenotypes are affected by variable parts of genomes. This applies equally to animals, plants and microbes, and cells, enzymatic and developmental systems.
The course centers on the theoretical and practical aspects of comparative analysis, about analyses of genomes, metagenomes and transcriptomes to study biological, medical and applied questions. The lectures cover structure and sequencing of genomes, transcriptomes and proteomes, molecular evolution, different types of bioinformatic data, shell scripts, intro to R and Python scripting and applications. The practicals include, retrieval of data from databases, blast and alignment, assembly and annotation, comparison of genomes, population data analyses. Students will work with databases, such as Flybase, Genebank and ENSEMBL. Data will be retrived with Biomart and Bioconductor, and data quality discussed. Algorithms for search tools and alignments, read counts and comparisons of groups and treatments. Also elements of python scripting, open linux software, installation of linux programs, analyses of data from RNA-seq, RADseq and genome sequencing.
Students are required to turn in a few small and one big group project and present the large project with a lecture. In discussion session primary literature will be presented.Face-to-face learningDistance learningThe course is taught if the specified conditions are metPrerequisitesTÖL604MAlgorithms in BioinformaticsElective course6Free elective course within the programme6 ECTS, creditsCourse DescriptionThis course will cover the algorithmic aspects of bioinformatic. We will start with an introduction to genomics and algorithms for students new to the field. The course is divided into modules, each module covers a single problem in bioinformatics that will be motivated based on current research. The module will then cover algorithms that solve the problem and variations on the problem. The problems covered are motif finding, edit distance in strings, sequence alignment, clustering, sequencing and assembly and finally computational methods for high throughput sequencing (HTS).
Face-to-face learningPrerequisites