Askja
Room N-132
Doctoral candidate:
Marcel Aach
Title of thesis:
Parallel and Scalable Hyperparameter Optimization for Distributed Deep Learning Methods on High-Performance Computing Systems
Opponents:
Dr. Marco Aldinucci, Professor at the University of Torino, Italy Dr. Matthias Feurer, Professor at the Ludwig-Maximilians-Universität München, Germany
Advisor:
Dr. Morris Riedel, Professor of Computer Science at the University of Iceland and Head of National Competence for HPC & AI.
Other members of the doctoral committee:
Dr. Helmut Wolfram Neukirchen, Professor of Computer Science & Software Engineering at the University of Iceland Dr. Andreas Lintermann, Leader Simulation and Data Lab Highly Scalable Fluids & Solids Engineering, Jülich Supercomputing Centre
Chair of Ceremony:
Dr. Rúnar Unnþórsson, Professor and Head of the Faculty of Industrial Engineering, Mechanical Engineering and Computer Science, University of Iceland
Abstract:
The design of Deep Learning (DL) models is a complex task, involving decisions on the general architecture of the model (e.g., the number of layers of the Neural Network (NN)) and on the optimization algorithms (e.g. the learning rate). These so-called hyperparameters significantly influence the performance (e.g. accuracy or error rates) of the final DL model and are, therefore, of great importance. However, optimizing these hyperparameters is a computationally intensive process due to the necessity of evaluating many combinations to identify the best-performing ones. Often, the optimization is manually performed. This Ph.D. thesis leverages the power of High-Performance Computing (HPC) systems to perform automatic and efficient Hyperparameter Optimizaton (HPO) for DL models that are trained on large quantities of scientific data. On modern HPO systems, equipped with a high number of Graphics Processing Units (GPUs), it becomes possible to not only evaluate multiple models with different hyperparameter combinations in parallel but also to distribute the training of the models themselves to multiple GPUs. State-of-the- art HPO methods, based on the concepts of early stopping, have demonstrated significant reductions in the runtime of the HPO process. Their performance at scale, particularly in the context of HPC environments and when applied to large scientific datasets, has remained unexplored. This thesis thus researches parallel and scalable HPO methods that leverage new inherent capabilities of HPC systems and innovative workflows incorporating novel computing paradigms. The developed HPO methods are validated on different scientific datasets ranging from the Computational Fluid Dynamics (CFD) to Remote Sensing (RS) domain, spanning multiple hundred Gigabytes (GBs) to several Terabytes (TBs) in size.
About the doctoral candidate:
Marcel Aach obtained his M.Sc. in Economathematics from the University of Cologne in 2021. Since 2021, he has been working towards his Ph.D. at the Jülich Supercomputing Centre and the University of Iceland with a focus on efficient Hyperparameter Optimization (HPO) for different scientific applications on High-Performance Computing (HPC) systems.
Zoom: https://eu01web.zoom.us/j/63936013802
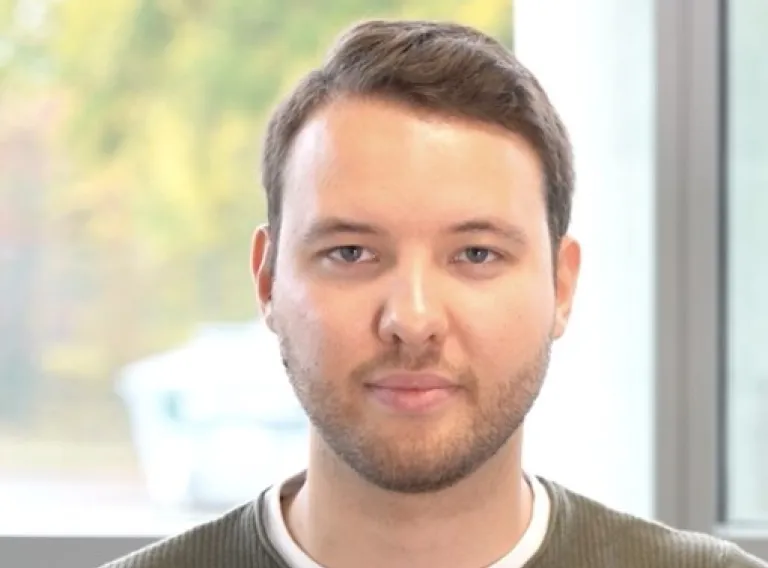
Share
Buses 14, 1, 6, 3 and 12 stop at the University of Iceland in Vatnsmýri. Buses 11 and 15 also stop nearby. Let's travel in an ecological way!